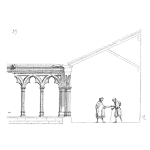
Part 1 of this article is here. And it covered Tips# 1 to 3.
AI projects are R&D initiatives and not simply IT projects. Organizations miss the mark in realizing value from AI as they undertake AI projects just like their network update, or systems infrastructure upgrade.
While both IT & AI projects use similar tools their objectives are vastly different. It is difficult to estimate an AI program’s results without first modeling the data on hand. We cannot claim 95% accuracy in predicting motor failure without first analyzing existing motor data. Developing a model needs iteration with various algorithms, hyperparameters, and processing techniques; the workflow is analogous to a research project.
How do you govern a research project? Let’s borrow some proven management tips from research labs.
4. Have a long-term ROI outlook
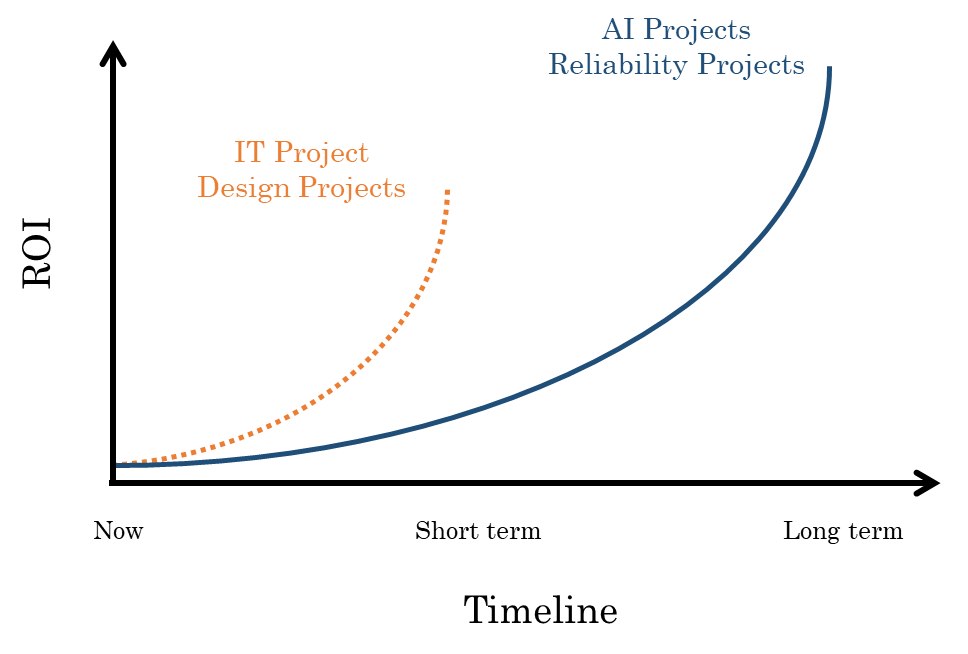
The iterative nature of the AI/ML workflow makes it time intensive. Most manufacturing industries are also not set up to have high-quality data today, so data pre-processing will take significant resources. Plan the project to accommodate a time frame that will allow it to demonstrate growth. Perform quick assessments before the start of the project and set cautious milestones.
Budgetary approvals from management require immediate ROI realization. Convey the gradual and longer ROI potential of AI projects to get approval for a longer time frame.
5. Iterate and improve continuously
Given the uncertainty in quantifying ROI, management might have an appetite for only a small-scale pilot. Start with a few assets and assess the performance of algorithms. For Predictive Maintenance, the model must be tuned to provide an accurate prediction that balances false positives while giving early warning of a failure. Algorithm KPIs must be defined & tracked to assess performance. The choice of algorithms to fit the data will depend on the resources available, user expertise, and requirement.
6. Scale up cautiously
Once a suitable model is developed, scale up across the sites to capture the results of an operation. Assets in industries operate under various conditions. A model developed for Condition#1 will give inaccurate results if deployed in Condition#2. One way to scale up across multiple use cases is to capture the conditions within the model.
Scaling up will require elastic computational resources and associated security improvement to prevent intrusions. Integrations with other systems and End-user identity management are other aspects to consider.
When managed effectively, AI projects like Predictive Maintenance will lead to value generation and a culture change in the organization. But as listed above, the pitfalls are many and not all firms can navigate them well. Bringing in external experts will guide your AI projects to success.
Owtrun offers turnkey Predictive Maintenance solutions to meet your maintenance & business needs. Our approach is founded on management principles & Asset Management. Contact us to get started on your AI journey.
Leave a Reply