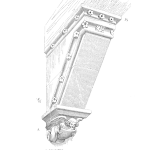
The history of Design of Experiments (DOE) can be traced back to the work of various individuals, including Genichi Taguchi, a Japanese engineer and statistician. Taguchi made significant contributions to the field, particularly in the area of robust design, which aimed to improve the quality of products and processes. His work was influenced by the need for quality improvement in post-World War II Japan. Taguchi’s methodology, known as the Taguchi methods, was based on the concept of “robust parameter design,” which aimed to make processes and products insensitive to environmental factors or other variables that were difficult to control.
The development of Taguchi methods was influenced by the work of Ronald Fisher, who proposed a methodology for designing experiments in the early 20th century. Fisher’s work laid the foundation for the development of experimental design techniques, which were later popularized by Taguchi. Taguchi’s methods, including fractional factorial designs and orthogonal arrays, were successfully applied and adopted by Japanese and Indian industries, and subsequently embraced by the US industry.
The main objective of Design of Experiments (DOE) is to systematically and efficiently study the relationship between multiple input variables in order to achieve the following purposes:
- Hitting a Target: Determining the optimal settings for key process input variables to achieve a specific target or goal.
- Maximizing or Minimizing a Response: Optimizing a response variable, such as maximizing product yield or minimizing defects.
- Reducing Variation: Identifying and reducing sources of variation in a process to improve consistency and quality.
- Making a Process Robust: Developing a process that is insensitive to variation in input variables or external factors.
- Seeking Multiple Goals: Balancing multiple competing objectives to find a set of input variable settings that achieve the best overall performance.
DOE is a powerful tool that allows for the manipulation of multiple input factors simultaneously, enabling the identification of important interactions and the effects of the factors on the desired output. By conducting carefully planned and executed experiments, DOE provides valuable information about the factors that control the value of a parameter or group of parameters, leading to improved process performance and quality.
The L8 matrix is designed to help identify the most significant factors affecting a process or product while minimizing the number of experimental runs. It is particularly useful for studying the effects of a small number of factors and their interactions on a response variable, as well as for reducing the impact of noise factors on the system.
A typical L8 matrix in Taguchi design is shown below:
In this matrix, the columns represent the control factors, and the rows represent the runs (combinations of factor levels). Each cell represents the factor level for that run.
The factors and factor interactions can be determined from the matrix. For example, in the L8 matrix above, the factors are A, B, C, D, E, F, and G. The interactions can be identified based on the columns that change between the runs.
When there are only three noise variables, the remaining columns in the L8 array can be used to estimate the interactions between certain noise factors.
The threshold of 2 levels means that each factor should be varied at 2 levels to study its effect on the response variable. The levels should be chosen such that they represent the typical range over which the factor is expected to vary in practice. This can be based on historical data, engineering knowledge, or other relevant information about the system under study. It’s important to note that the choice of factor levels should be based on the specific context of the problem and the goals of the experiment. The levels should be chosen to provide meaningful information about the factors’ effects on the response variable, while also being feasible to implement in the actual system or process.
For example, one of outcome the results can looks like follow:
H6 – is the signal that has major impact, H1 is next in ranking then H2,H3,H7, and almost no impact it is H4, H5.
In summary, DOE provides a more comprehensive and efficient approach to studying and optimizing processes or products, leading to improved quality, reliability, and performance compared to traditional testing methods
Leave a Reply