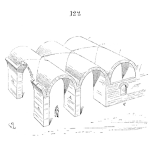
and Probably Already Has
Predictive Maintenance strategy uses condition monitoring techniques to observe plant and equipment health. Based on the equipment’s condition you plan, schedule and perform any necessary maintenance before the breakdown. To use Predictive Maintenance most successfully you must set-up and run your condition monitoring program correctly all the time—predictive maintenance is a never ending strategy that if not done right at every step will still lead to plant and equipment failures. Learn about the many ways your predictive maintenance program will fail and you won’t even know about it!
Keywords: predictive maintenance, condition monitoring, Con Mon
In our 2-day Root Cause Failure Analysis training course we run a team activity to practice doing RCFA. People are given an engineering report on the failure of an electric motor drive end roller bearing. It includes the following plot of the weekly temperature reading from the roller bearing. They are required to find the root cause of the 27th July bearing failure.
Only once in all the years we have delivered the course has an RCFA training team got the answer right. But the machine bearing failure is not what is really important about the activity to take away and imbed deeply in your mind and into your company’s collective knowledge
Figure 2 is a screen image straight out of a lubrication condition monitoring report. It’s from a company in Canada. The same horrible problem as is shown in Figure 1 is also evident in that image. In fact, there are a number of very serious failures in this company’s use of Predictive Maintenance (PdM). They do not yet know it, but their PdM program is seriously failed and they will continually get totally preventable failures. Can you ‘see’ all that is wrong with their condition monitoring program? Look past the obvious bad results and spot the deeper failures.
I could tell you what the problems are and you would simply nod your head and agree with me. But agreeing with me is pointless—you must go and see if you have the same problems already happening in your company; and then you must fix them if they exist!
Instead of me telling you what I ‘see’, I’m going to ask you to tell me what you ‘see’.
Let me know what you think I find is horribly wrong with the plot in Figure 1 and the information in Figure 2. I’ll give the first 10 people who get the list right, and explain to me why they put the items in their list, a free, signed copy of my book, Plant and Equipment Wellness (Way).
Eventually I’ll publish the first ten correct responses at the bottom of this white paper. If you want your name, company and your position to be included in the first 10 published comments, then tell me all that you see wrong in those figures and email your list and reasons to me.
Who Must Have the Ability to ‘See’ these Problems in a Condition Monitoring Program?
Every person who offers maintenance management or asset management consulting services needs to immediately be able to ‘see’ the deeper problems in Figures 1 and 2. As must every person who holds a position higher that Supervisor in an organisation’s engineering management, maintenance management, asset management, reliability improvement, Lean or Six Sigma groups. If these people cannot ‘see’ the problems by simply looking at the two figures; their education is lacking!
You can get our email contact details from the LRS Consultants Global website www.lifetime-reliability.com.
All the very best to you,
Mike Sondalini
LRS Reliability Consultants
Some problems with the PdM:
1. Data collection frequency is too low- allowing failures to manifest within the time.
2. Parameter monitored might not be the best indicator. There are other parameters that might be a better “failure indicator” than Temp or Particle count.
1. Technique is wrong. Putting your trust on thermal survey for high speed rotational elements will prove to be unfruitful as this symptom manifest at a point way to close to functional failure, and degradation is fast. Vibration analysis would be a better fit.
2. Measurement frequency is not respected, as there is one analysis in 2009 and another in 2013.
Anyway, no proper understanding of cause can be done solely relying on the shown data. You always need to judge them in the context of the historical interventions in the machine, the operational regime and the environment it is inserted into (and presuming that data collection has been done according to best practices)
Problems in the 1st case:
– The abrupt temperature rise (with no decrease) of the bearing could indicate that some overload (stress) happened, due to variabilities in the operational processes, and caused a step change in the bearing strength, that accumulated some wear, and increased its failure probability.
This variability induced by human factors cannot be addressed by predictive maintenance programs due to its random nature, i.e., no consistent degradation. So monitoring the temperature, although useful in some other applications, may not be effective in this case.
Problems in the 2nd case:
– Lack of compliance with the oil sampling frequency.
Once again, this could indicate variabilities in the processes. Not sampling and possibly renewing the machine’s oil can allow wear to accumulate and increase the risk of failure.
But I see other problems related to the reports specifically that I think are common in both cases.
– No trends in the data to visualize degradation speed and estimate future conditions.
– No targets setting actionable levels to motivate when intervention is needed.
– No limits to define acceptable and unacceptable ranges.
Without these characteristics we can have only some plots that are not an informative or action-enabling.