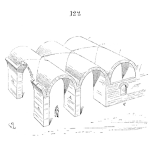
Well, this article will be a little bit challenging and full of statistical terms. I would categorize the “Hypothesis testing” as most of common use in statistical analysis.
First time of usage happened in 1700s, but actual popularisation at early 20th century:
First thing first let’s see how Wiki define it:
A statistical hypothesis test is a method of statistical inference used to decide whether the data at hand sufficiently support a particular hypothesis. Hypothesis testing allows us to make probabilistic statements about population parameters. (Source Wiki)
Having read that few times, this definition, still leaving us in fog, what all this about?
The hint is in Hypothesis, there is total 2 of those:
Null Hypothesis (H0) stating that there is no significant difference between a hypothesized value of a population parameter and its value estimated from a sample drawn from that population, or in other words “whatever is you trying to do is not changing anything”.
You change value of pressure in tube, but sensors showing you exact the same value as before the change.
Alternative Hypothesis (H1) stating that there is a significant difference between a hypothesized value of a population parameter and its estimated value. Whatever change you do, is having an effect.
You are measuring change of temperature as reaction to increase power in heating element.
Possible outcomes of those Hypothesis:
Reject H0: we are saying that whatever you trying to do, have different results from previous known observations. (Mean H1 is true)
Fail to Reject H0: we are not having enough evidence to say that the change has any impact, and it is the same as was observed before. (Mean H0 is true)
But how we can be sure, that we rejecting or failing to reject is right?
And here come into the picture Statistical significance (also called Probability value (P-value)) or Confidence level – where we tell ourselves, yes this has very high chance to occur and lead us to decision.
Confidence level (CL) and Statistical Significance (a – alpha) are dependent in the next way
a = 1 – CL
In the next example let’s apply the hypothesis testing.
In one high school in Canada, was chosen to test claim about usage of mobile device during the lessons and if it has negative impact on final average grade. For those purposes was chosen two random population of students, 24 students that not allowed to use any mobile devices, and 28 students, that was completely free to use during the class time.
And we want to be confident in our hypothesis test at 95% confidence level, or with p-value of 5%.
And the results (thanks to GraphPad)
Having P-Value = 0.0744, giving us confidence of 1-0.074= 92.6%. Meaning it is 92.6% chance that using mobile device lead to different average than in the group, that not using mobile during the lessons.
If we stick to request of 95% Confidence level (or P-value 5%) means we fail to reject Null Hypothesis.
So by the end of the day, it is up to us have a decision and understanding in the process will give us confidence.
Want to know more? Ask in comments below!
Leave a Reply