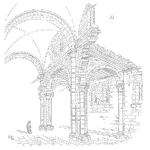
Hi Fred,
I would take this opportunity to ask the reliability guru about bathtub curve for hardware reliability. I am running 27 units for life test for a million cycles around 555 hours. I have one failure at 300,000 cycles, and the rest of the units are running fine. Would this be classified as an early life failure? Also, how do I make a determination of when the early life failure time interval ends and constant failure rate starts in this example based on failure rate of remaining units? Thanks.
–and my response —
You have two next steps. Continue to run the testing till you have more failures and plot the results. The units that have not failed are censored, yet you do need enough failures to determine the slope and distribution fit (at least five).
Second, determine the root cause of the failures. If it is a wear out mechanism, like solder fatigue, then it’s pretty safe to say it’s wear out. IF it’s a poorly assembled unit, you may lean toward early life failure.
In the best case, you’ll experience the same failure mechanism for each failure. This permits you to use published literature about the failure mechanism to confidently fit a life model to your data. If each failure is different, then modeling at the system level and using only an empirical or non-parametric fit may provide some information about the expected performance, yet it will be difficult to assign an acceleration factor to the results.
Keep in mind that there are many ways a product can fail and they do not arrange themselves into convenient groups. In a life test, you most likely have a specific stress that is being applied that will excite the acceleration of only a subset of possible failure mechanisms.
Good luck with your testing and data analysis.
Cheers,
Fred
On 03/11/12 8:10 AM, Kartik Ramaswamy wrote:
——————–
Hi Fred,
I would take this opportunity to ask the reliability guru about bathtub curve for hardware reliability. I am running 27 units for life test for a million cycles around 555 hours. I have one failure at 300,000 cycles, and the rest of the units are running fine. Would this be classified as an early life failure? Also, how do I make a determination of when the early life failure time interval ends and constant failure rate starts in this example based on failure rate of remaining units? Thanks.
Related:
Sources of Reliability Data (article)
Reliability Management Terminology (article)
Failure modes and mechanisms (article)
hello fred,
One question here…pertaining to your above response. all said above is right..but one aspect i felt was more required was why the sampling considered as 27 units? I think this will also have an impact.
please correct me..
regards,
prabhakar
Hi Prabhakar,
27 units for a sample size may or may not be adequate – it really depends on what sampling risk you are willing to take. I’m a statistician and would always like to see more samples, yet the reality is we often have budget, space, or other constraints. I have found that about 20 samples if often good enough to estimate a distribution when there are plenty of failures. If I’m making assumptions on the failure mechanism and acceleration model – we often run a test expecting no failure unless the unknown true failure rate is above some threshold – the sample size really doesn’t help with those assumptions, yet it is common practice.
27 isn’t a magic number – it’s not too low, yet depending on the details of the test design, expected variation, and tolerance for risk that the sample doesn’t represent the population.
cheers,
Fred
Fred,
Why a minimum of five failures to determine slope and fit? I’ve heard three before, but only as a rule of thumb.
Dave Wakefield
Hi Dave,
Actually if you ask a random group of statisticians you’ll get a different answer from each on average.
One failure point and an assumption on the shape parameter is done – with risk or the assumption being wrong.
Two failure to fit a straight line and risk of the slope be very, very wrong.
three failures to fit a line and check for curvature – absolute minimum and generally risky
5 out of 20 samples provides information about the lower quartile – while more failures and more samples would be great – it’s a trade off on time, testing costs, sample costs, and the early estimate with less risk then having less information.
Cheers,
Fred
Fred,
Thanks for the great explanation. Pretty obvious now that I’ve thought it through!
Respectfully,
Dave