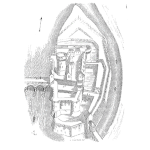
In this article post, we discuss the suggested steps for planning a Designed Experiment.
Step #1 – Clearly Define the Problem and Objectives
It is critical to clearly define the problem before beginning experimentation. When the problem is not clearly defined and described, there will be confusion in designing and executing effective studies. To define appropriate responses to measure requires that the problem be understood and agreed on. Also, it is key to define the objectives of the actual experiment. If the problem is to reduce scrap rate, how much of a reduction is targeted?
Step #2 – Select the Team
Design of Experiments rely on lots of different subject matter knowledge, experience, intuition, and perspectives from those affected by the problem we are trying to solve. It is important to consider all sources of variation and contributors to a problem so it can be determined what should be included in the experiment. Consider a team that includes design engineers, process engineers, manufacturing engineers, operators, maintenance staff, scientists, statisticians, black belts, customers, supplier personnel, and anyone else that may provide insights.
Step #3 – Identify Response(s)
Recall that responses are the outputs that are measured during an experiment. Clearly, the response should reflect the presence or degree of the problem we are trying to address. For example, if we are trying to prevent or minimize leaks from a valve, then perhaps the response would be leak rate.
Because the DOE may suggest design or process changes, it is important to consider all relevant responses that might be affected even if some of them are not related to the problem at hand. The last thing we want to do is fix our issue yet introduce additional issues because the changes made to fix the first issue.
In general, the effort in DOE comes from performing all the various experimental combination setups and measuring some additional responses often will not be too costly. Very often, product performance measures involve tradeoffs and our solution should optimize the whole system rather than just one attribute. Having the important responses allows models to be developed for each response and multi-response optimization can be done to find that “sweet spot”.
Step #4 – Ensure adequate Measurement Systems
Measurement Systems must be able to evaluate and measure the responses accurately and consistently for the results to be useful. We have seen many experiments produce worthless results due to inadequate measurement systems. Many techniques are available for assessing measurement systems such as Gage R&R studies, Accuracy studies, Linearity studies, etc. In our consulting practice, we require evidence of adequate measurement systems before we provide a protocol to our clients for conducting the experiment. This is critical.
Step #5 – Identify Factors for the DOE
The team must determine what factors should be varied in the study. The number of factors will affect the types of studies that may be performed and the effort required. It is recommended to use brainstorming techniques to identify a comprehensive list of all possible factors that are believed to affect the key responses. Often the list must be prioritized and narrowed to a reasonable number (often no more than 5-7 factors). Factors that do not make the cut should be held constant during the study and the results will be in that context.
Step #6 – Identify the Factor Levels
How much should the factors be varied? What should be the highest and lowest values? This step is often more an art than a science. It is important to push beyond our comfort zone and consider levels that may be outside of historical practice. After all, this is our opportunity to learn about the process! Also, to identify statistically significant results, we need to ensure that the factors changes produce enough variation in the responses to overcome the natural error/noise in the data due to uncontrolled sources of variation. In our experience, many people tend to be too conservative in the range of factor levels. The goal is to see LOTS of variation in the output so that we see the effects. It’s useful to produce some good stuff and some bad stuff. The number of levels is also important and that leads us to the next step.
Step #7 – Select the Experimental Design
In and earlier article post, we discussed the evolution of experimental design from screening to optimization. Depending on the number of factors we start with, we need to define the type of study to run. Options include fractional factorial designs, full factorial designs, central composite designs, etc. We will discuss these types of designs in future articles. It’s important to use our resources wisely and consider the phase of experimentation that is most relevant for our situation.
After the planning is complete, we are ready to conduct the experiment. There are some important aspects of conducting the experiment and these will be discussed in the next article.
Leave a Reply