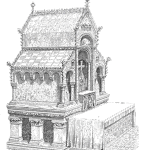
Generally, I do not talk about statistics before 10am – it’s not polite.
As a reliability professional, statistics is a central feature of the value I bring to the team. And, not just the reliability statistics, all stats. It continues to amaze me how many engineers, scientists, and professionals tend to avoid statistics.
Ok, maybe those of us that enjoy working with variability and understanding the ways to statistics are not normal (pun intended). In some way, that also makes us valuable. Conducting a hypothesis test, using design of experiments to isolate a failure mechanism, designing an accelerated life test are all examples where understanding and using statistics is part of our role.
Understanding process variability, control charts, and capability indices not only help us improve yield, but it also provides meaningful information to create ongoing reliability tests or work with vendors to improve their processes and stability. Use the data to make decisions.
Understanding the environmental range is nice, detailing the distribution of the environmental factors is great. Understanding the use profile limits is necessary, yet knowing the distribution of expected use is better. Derating, stress strength, and product reliability test design all hinge on using the best available data about the expected environment and use profile.
Reliability engineering is not only facilitating an FMEA or calculating a parts count prediction. Reliability engineering is about making decisions. The use of statistics as a tool is confined to reliability professionals as you know, yet you are often the professional in the building that both needs to understand the design’s ability to withstand material and process variation, and has the knowledge to discuss variation. Statistics.
One of the disservices of undergraduate statistics is the extensive use of the Gaussian (normal) distribution. The mean, median and mode are the same and at the 50th percentile unless the distribution is skewed (slanted to one side) and it is symmetrical. For most distributions useful for reliability work, they are not symmetrical, and the mean is not at the 50th percentile.
For example, the exponential family of distributions (exponential, Weibull, etc.) has a mean value at roughly the 63rd percentile. Therefore, one considering the average value for some field returns, using the same basic calculation as for a normal distribution does not yield the same kind of number we experienced when using the normal distribution.
The mean, median and mode are terms used to describe the central tendency. We are assuming the data is not along some predefined pattern or grid. For example uniformly spread out along the number line from zero to 100 at intervals of 10, with an equal count at each of 0, 10, 20, etc. (that would be rather boring and rarely seen in nature, uniform distribution.) We assume the many and varied causes of variation tally to something like the familiar normal distribution. Something with a relatively smooth increase in expected counts till a maximum and gentle and smooth decrease on the other side. We’re not expecting multiple peaks, sharp or sudden changes, etc. With enough data, based on measurements or field returns, we almost always see the histogram (pdf) as a smooth curve. It is the mean, median and mode that describe the central or peak of that curve.
The mean is the center of gravity of the data. Half the data points are above, and half is below. If each point had some mass, and we placed the points along a beam, we could balance the beam at the point called the mean.
The median is the middle of the rank order of the data. Sort the data points from lowest to highest and count to the middle or midway of the number of data points that is the median. There are simple rules for an odd or even number of points, yet this calculation does not take into account the weight of the points (meaning the data’s actual value).
The mode, which is rarely used outside Pareto plotting, is the most common data point value. For example, in a simple data set that looks like this: 3, 4, 5, 3, 6, 7, 3, 8, the mode is 3, as it appears in the dataset three times whereas they others only appear once each.
Those are a couple of basic definitions, and I tossed in a couple of other terms to be defined fully in later posts.
Related:
Basic Statistics (article)
Sources of Variation (article)
Why do statistical based testing? (article)
Your comment about engineers shunning stats methods results from knowing management(their boss) doesn’t know probability theory and how its used in decision making. Therefore why bother. AS Dr. Deming said 80% of the problem lies with the system not the operator.
In my experience (30 +years) as a statistical engineer , including attempting to teach stat to engineers, results were poor.
Successful use of stats methods was done by the Six Sigma folks which became a huge business and is taught by many organizations. You are correct they use the normal curve extensively.
Attempting to break in this culture with rel eng methods presents another challenge.
My suggestion for you is find a way to tag on to six sigma.
FYI I believe the best book on rel methods is Wayne Nelson “How to Analyze Reliability Data’ a ASQC publication.