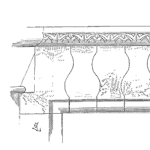
Stratification implies layers or differences. A quick test for soil composition is to place a sample of soil with water in a clear jar and give it a shake. The sand, silt and loam will settle at different rates and create a layered appearance within the jar over time. The height of each layer provides information about the proportion of each type of soil within the sample.
Stratification as one of the seven basic quality tools (some lists use a run chart or flowchart instead) the idea of layers or differences still applies. The idea is to identify potentially meaning differences within a sample set.
Reasons to Use Stratification
When we are faced with a set of data we often want to understand the information contained within the dataset. A common technique is to plot the data using histogram or scatter plot. Yet at times, those are not informative. about potential underlying common causes of differences within the data.
The primary reason to use stratification is to learn a little more about the data. For example, what causes the higher/lower values? What separates good items from out of spec items? What are the significant sources of variation within our process? and so on.
We may be exploring difference, and want to initially detect if a difference exists, between shifts, different factory lines or locations, different materials, amount suppliers for a similar part, time of day or week, seasonal differences, etc.
Identifying differences that relate to some factor may allow eliminating or reducing the variability. Or, it may identify a potential root cause source for unwanted variability. Or, the identified differences may lead to calibration, measurement system, or alignment problems within the system.
Stratification can also identify new product segmentation or solutions for customer problems.
In short, stratification is a tool that allows us to better explore and understand data.
The Basic Stratification Process
Back to the soil testing process, if presented with a dozen soil samples and their testing results, what additional data would be useful? Maybe the location and depth of the sample source? How about a description of the local environment, such has ocean beach, temperate forest, or grasslands?
If possible, when collecting samples, note the salient aspects or conditions for each sample. What data characteristics or conditions may provide meaningful information for the understanding of the dataset.
If the samples do not arrive with additional information, the process to find the various characteristics may take a bit more work. For example, when noticing a spike in failures for an op amp, you may be able to find (if product tracking serial numbers and lot number tracking for components data is available) a bit more information. Such as:
- location and type of use or test when failure occurred
- age or operating hours
- location of the part within the system, if more than one possible
- assembly date and location
- was the component replaced or was a nearby component replaced
- week of manufacture or lot/batch for specific components
- and so on.
The next step is to look for patterns or similarities. For small sets of data this may become obvious with simple inspection. For larger datasets plotting with different colors or markers for different characteristics values may be insightful.
Finally, conduct further analysis of subsets for the different layers identified. For example, do components that have been replaced experience a different assembly handling or set of stresses that may causes internal latent defects, versus those that did not incur replacement?
Pitfalls and Considerations
Years ago I heard a short segment on the Radio Lab show, that described how Mary Snow for a science project released a helium ballon with a not requesting information on where the ballon was eventually found. When a week later it was found by a different Mary Snow, that was an interesting event. The reporter than mentioned that both Mary’s were in the 5th grade, enjoyed science, both liked ponies, the color green, and both of their fathers are named John. The report went on to list a dozen additional similarities between the two Marys.
What the Radio Lab segment explored was the long list fo similarities was really just a subset of a much longer list of possible questions the reporter asked. For example, one like playing soccer while the other did not. The list of differences was likely much longer than the similarities.
Gathering a lot of data for exploration using stratification will find apparent stratification when none actually exists due to simple random chance. When gathering data, gather that data which has some rational reason related to noticed differences.
Another issue is using stratification to assign the root causes. Just because all the failed components were from the same lot for the same manufacture doesn’t guarantee that that lot is bad. All the noticed failures may be due to a faulty assembly process that occurred when that lot was part of the assembly process.
The information provided by the use of stratification provides clues for an explanation for the anomalies. Don’t skip the last step. Be sure to analyze, experiment, examine, etc until there is a clear understanding around how the stratification observed was actually created.
Stratification is a useful tool as we work to understand the world around us. It’s not perfect, yet may be informative.
Who implemented that stratifican tool of quality, and when was it
HI Amos,
I’m not sure, nor sure anyone has claimed the invention of any of the 7 basic tools. One reference lists the seven tools, Ishikawa 1985, yet doesn’t go into the history or invention. To your question of who implemented these tools, well Ishikawa certainly did, as have I, on many occasions. One of the primary reasons to always plot the data is to check for conditions that suggest stratification will be informative.
cheers,
Fred