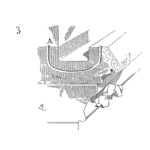
The t-test was first described by William Sealy Gosset in 1908, when he published his article under the pseudonym ‘student’ while working for a brewery. Gosset, an Englishman, developed the t-test and t distribution, and he is considered the “godfather” of the t-test.
Sir Ronald Aylmer Fisher, another prominent figure in statistics, is known as one of the founding fathers of modern biostatistics, but the t-test is attributed to Gosset’s work.
The t-test is a statistical hypothesis test used to determine if there is a significant difference between the means of two groups. It is commonly applied when the test statistic would follow a normal distribution if the value of the scaling term is unknown. There are three forms of the t-test: the one-sample t-test, the two-sample t-test, and the two-sample paired t-test.
- 1-Sample T-Test
The 1-sample t-test is used to determine whether the mean of a single sample is significantly different from a known or hypothesized population mean. For example, it could be used to test if the average weight of a sample of apples is significantly different from 150 grams.
- 2-Sample T-Test
The 2-sample t-test is used to compare the means of two independent groups. For instance, it could be applied to assess if there is a significant difference in the average test scores of students who used two different study methods.
- Paired T-Test
The paired t-test is used when the observations are not independent, such as when the same group is measured at two different times or under two different conditions. For instance, it could be used to determine if there is a significant difference in the blood pressure of individuals before and after a treatment.
The t-test is not recommended for data with severe outliers, categorical variables, paired measurements, or when the data do not meet the assumptions of normal distribution and unknown variances. In such cases, alternative statistical tests should be considered.
Some of the alternatives to the t-test include:
- Z-Test: A z-test is used when the sample size is large, and the population standard deviation is known. It is similar to the t-test but is based on the standard normal distribution.
- Chi-Square Test: The chi-square test is used to compare categorical variables. It has different variations, such as the goodness of fit test and the test for independence, and is suitable for analyzing categorical data.
- Analysis of Variance (ANOVA): ANOVA is used to compare the means of three or more groups. It is an extension of the t-test for multiple groups and can help determine if there are statistically significant differences between the means of the groups.
- Wilcoxon Rank-Sum Test: This non-parametric test is an alternative to the t-test when the assumption of normality is violated or when the data is ordinal rather than interval.
These alternative tests provide options for different types of data and research questions, allowing researchers to choose the most appropriate statistical test based on the specific characteristics of their data and the nature of the comparison being made.
Leave a Reply