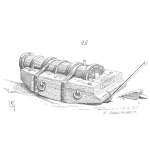
There is a type of error when conducting statistical testing that is to work very hard to correctly answer the wrong question. This error occurs during the formation of the experiment.
Despite creating a perfect null and alternative hypothesis, sometimes we are investigating the wrong question.
Example of a Type III Error
Let’s say we really want to select the best vendor for a critical component of our design. We define the best vendor as their solution or component is the most durable. Ok, we can set up an experiment to determine which vendor provides a solution that is the most durable.
We set up and conduct a flawless hypothesis test to compare the two leading solutions. We can very clear results. Vendor A’s solution is statistically significantly more durable than Vendor B’s solution.
Yet, neither solution is durable enough. We should have been evaluating if either solution could meet our reliability requirements instead.
Oops.
Even if we perfectly answer a question in our work, if it’s not the right question, then the work is for naught.
Short History of Type III Errors
Neyman and Pearson used the terminology for type I and II errors as “error of the first kind” and “errors of the second kind”, respectively. This led others to consider other types of errors, naming them “errors of the third kind” and so forth.
An occasional college of Neyman and Pearson, F.N. David, in a paper published in 1947, suggested she may have a need to extend the Neyman and Pearson’s sources of error to a third source by possibly “choosing the test falsely to suit the significance of the sample.”
Mosteller in 1948 defined Type III error as “correctly rejecting the null hypothesis for the wrong reason.”
Extending Mosteller’s definition, Kaiser in 1966, by defining such a Type III error as the coming to an “incorrect decision of direction following a rejected two-tailed test of hypothesis”.
Kimball, in 1957, suggested a definition close to how I consider a Type III error, as “the error committed by giving the right answer to the wrong problem”.
and so on…
There is no one widely accepted definition for an error of the third kind nor for Type III errors. Yet, for any of the above definitions, the error is one to guard against making by careful consideration when designing, conducting, and analyzing statistical tests.
A Few Pitfall Situations that Lead to Type III Errors
An obvious situation, in hindsight, is the experimenter solving the wrong problem or asking the wrong question. The cause here could be simple ignorance of sufficient information to recognize the error. Another cause could be focusing on the first or most interesting question to investigate.
Another set of situations may be the deliberate or unconscious effort to connect the experimental results to an expected outcome. This sometimes occurs when reinterpreting the results when the results do not agree with the desired outcome.
Another set includes the process of just doing what we always have done. In this case, the experimenter may not even have a connection between the experiment and a suitable hypothesis that would enable analysis. We can do a test or experiment perfectly well, yet it has no meaningful result or influence on any future work.
Yet other situations exist. If you spot one or more that I missed, please add your thoughts in the comment section below.
Prove any null hypothesis!
Restrict the alternative hypotheses to unlikely possibilities and you can prove practically any null hypothesis. A typical application of this cheat that I learned in school is to test constant failure rate against the alternative of an increasing failure rate. That alternative hypothesis leaves out failure rates that may increase for some ages and decrease for others, a more likely alternative hypothesis than a monotonically increasing failure rate.
This is an example of Type III error, population misspecification. Ronald Fisher recognized the job of a statistician as:
1. specification of the kind of population that the data came from
2. estimation
3. distribution specification.
By specification, Fisher meant that the statistical distribution(s) involved should encompass both the null and the alternative hypotheses.