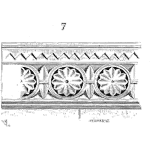
A simple assumption in many experiments is to assume the variable act independently on the response.
This means when I change the temperature a little in a polymer dryer silo that time to achieve a certain dryness goes down. And, changing the humidity or airflow rate or pressure either do not change or as they change has no impact on the relationship between temperature and drying time.
Hold on, even those not with little knowledge of polymer drying are probably saying, temperature, pressure, etc are most likely related and in certain combinations will impact the drying time differently.
The set of variables may combine or interact to create a new variable that we should consider.
A simple DOE
When first learning about design of experiments we often learn about the simple example of three factors (variables) each with two levels (settings).
We can run 4 carefully constructed experiments and learn which of the three factors is causing the most change in the response. By design, we assume the three factors do not create any meaningful (significant) change to the response that is different than the change wrought by three factors under consideration.
In the polymer drying example, we may well suspect some impact of interactions, and the changes to the response are going to occur.
So, where do those results appear? And, is it possible to detect the interaction results?
We can answer the first question based on the design of the experiment. The second question is no, not unless we specifically take steps to measure the interaction term.
Confounding
Confounding is when the effects of two or more factors are not separable.
A factor may be the combination of two factors. For example, temperature and airflow rate may combine to create a new factor that as the temperature and airflow product changes the result changes which is separate from the impact on the result of just temperature or just airflow rate.
In the example of three factors in a simple DOE array, we would assign each factor to a column. Say temperature to column A, airflow rate to column B and humidity to column C. Given a Taguchi L4 design, the product of A and B is the interaction term for A and B and will add its influence to column C. Thus the response associated with humidity (column C) will include the response caused by the interaction of the temperature and airflow rate.
It is this mixing of results that we are unable to separate that is confounding.
Seeing Interactions
If we suspect that temperature and airflow rate may have a combined effect on the response, then we can create a design that does not include humidity in column C.
Instead, we assign temperature to column A and airflow rate to column B and assign the interaction term AB to column C. In the creation of the experiment the AB term is a factor. And the analysis will provide the response associated with AB without being confounded with humidity since humidity is not part of the experiment.
Each DOE design will have some amount of confounding thus it is important to consider interaction terms and where the response due to those terms will appear in the analysis.
Treating known or suspect interactions as factors permits you to avoid confusing results in your experiment.
Related:
Design of Experiments (article)
Second 5 Questions (article)
Three considerations for sample size (article)
Leave a Reply