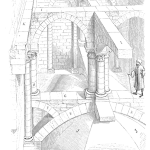
Someone asked, “…if you can give me quick explanation: For Example, EPRD 2014 part, Category: IC, Subcategory: Digital, Subtype1: JK, Failure Rate (FPMH) = 0.083632 per (million) calendar hours! How do you convert that to operational hours?” I.e., time-to-failure T has exponential distribution in calendar (million) hours with MTBF 11.9571 (million) hours.
Did the questioner mean how to convert calendar-hour MTBF into operating-hour MTBF? David Nichols’ article does that for 217Plus MTBF predictions, based on “the percentage of calendar time that the component is in the operating or non-operating (dormant) calendar period, and how many times the component is cycled during that period.” I.e., MTBF/R where R is the proportion of operating hours per calendar hour.
There could be reasons for converting to operating hours. For example, A “War Readiness Spares Kit” [Glazener and Tinder] recommends spare parts required to support a flying-hour program plan in case of war and deployment away from the spare parts’ inventory. The flying-hour program specifies aircraft war-time operating hours, so an actuarial parts demand forecast requires their actuarial failure rates in operating hours [Hess, Hogge, Kamins and McCall, Long and Engberson].
What if the Proportion R is Random?
Suppose the operating rate R = operating hours per calendar hour has a uniform distribution on [a, b] with 0<a<b<1. The failure rate function of operating time-to-failure, X=T*R (T = calendar-hour time to failure), is NOT constant; it is toonasty a formula for publication here. The formula was computed using Mathematica.
Figure 1 graphs the failure rate function from 1100 simulated times to failures in operating hours. The calendar time-to-failure T has exponential distribution with constant failure rate of 0.08362 per (million) hours, and the proportion of operating time R has uniform distribution between 0.25 and 0.75. The formula in figure 1 is for a quadratic-polynomial trend line, an approximation to the nasty formula. The non-zero coefficients for the quadratic and linear terms are not zero indicating operating time failure rate is NOT constant.
![Figure 1 is the simulated (1100 repetitions) failure rate function in operating-hours with operating hours per calendar hour R distributed uniformly on [0.25, 0.75].](https://s3-us-west-1.amazonaws.com/accendo-media/wp-media-folder-accendo-reliability/wp-content/uploads/2023/05/ConFig1.png)
For this example, the operating time failure rate function of T*R changes from 0.180306 (at age 1) to 0.15665 (at age ~10 (million) operating hours). The operating-time failure rate at age 1 is about twice the calendar time failure rate initially because there is a 50% chance of operating in the first calendar period.
The MTBF in operating hours is 11.957*(a+b)/2 = 5.98752. (11.957 is the calendar-hour MTBF of an exponential distribution with (constant) failure rate 0.083632.) Nichols’ article says MTBF in operating hours is MTBF(calendar)/E[R]=MTBF(calendar)/((a+b)/2). That’s probably not true for other distributions of T, times-to failures, and R, operating hours per calendar hour.
“Constant Failure-Rate Models may be Hazardous to your Design” [Bowles, ReliaSoft]. Age-dependent failure rate (FR) function means the simple series system MTBF prediction, 1/(1/FR1 + 1/FR2 + … + 1/FRk), for k independent components, is wrong! What if a system is not in series? What if not all parts have same distributions of operating hours/calendar hours? What if parts’ operating hours/calendar hour are differently distributed, statistically dependent, or all of the above? [Schenkelberg]
Alternatives
- Use the MTBF software and constant failure rates to compute MTBF in calendar time and convert it to MTBF/E[R] (E[R] is expected value of R.) Warn customers that that formula is correct only if calendar-hour failure rate is really constant, and if operating hours per calendar are uniformly distributed!
- Send data on parts, their failure rates (calendar hours), and data on their operating hours/calendar hour to pstlarry@yahoo.com. I will estimate the distribution of R and plug it into an Excel workbook or Mathematica, and send back the inconstant failure rates per operating hour. If you send system reliability block diagram, I will (try to) compute or simulate system reliability and MTBF.
- Use MTBF prediction software as if operating-hour failure rates are constant/E[R], E[R] = the average operating hours per calendar hour, so that you can compare your lies with others’. Warn consumers of your operating-hour MTBF predictions and of your assumptions, explain the consequences, and ask for data on operating hours/calendar hour.
- Give up on MTBF predictions based on untenable assumptions and use field reliability data to estimate reliability and MTBF, or, if you’re trying to predict for a new product without field reliability data, ask for “Credible Reliability Prediction” [George], because it shows how to use field reliability of previous generations of products and parts.
Conclusions
Converting constant calendar-time failure rate, with random operating time per calendar-time unit, yields inconstant an operating-time failure rate function. That invalidates simple reliability and MTBF predictions that depend on constant failure rates.
Send data on field reliability and operating rates per unit time (please describe) to pstlarry@yahoo.com, and I will send back nonparametric field reliability and failure rate function estimates, operating rate distribution estimates, and convert between operation and calendar time scales.
If you want the failure rate function per operating hour for non-exponential time-to failure in calendar hours or R = operating hours/calendar hour with a non-uniform distribution, let me know. Send samples of operating hours per calendar hour or specify distribution of R.
I wonder how EPRD came up with (constant) Failure Rate (FPMH) = 0.083632 per million calendar hours for an IC?
References
Bowles, John, “Caution, Constant Failure-Rate Models may be Hazardous to your Design,” IEEE Transactions on Reliability, Volume: 51, Issue: 3, Sept. 2002, http://ieeexplore.ieee.org/document/1028412/
L. L. George, Credible Reliability Prediction, 2nd Edition, August 2022
Charles U. Glazener and Alan J. Tinder, “War Readiness Spares Kit Composition: A Systems Approach,” AFIT/SLSR 30-76B, Air Force Institute of Technology, AD A032426. Nov. 1976
Hess, Tyler, “Cost Forecasting Models for the Air Force Flying Hour Program,” AFIT/GCA/ENV/09-M07, Air Force Institute of Technology, http://www.dtic.mil/dtic/tr/fulltext/u2/a499658.pdf, March 2009
Hogge, Lewis J., “Effective Measurement of Reliability of Repairable Air Force Systems, AFIT/GSE/ENV/12-S02DL, Air Force Institute of Technology,https://pdfs.semanticscholar.org/a005/9387c34417acc0a6223be7eaed2dbb3d9476.pdf, September 2012
Kamins, Milton, and J. J. McCall, Jr., “Rules for Planned Replacement of Aircraft and Missile Parts, RAND Research Memorandum RM-2810-PR, November 1961
Long, William S. and Douglas H. Engberson, “The Effect of Violations of the Constant Demand Assumption on the Defense Logistics Agency Requirements Model,” AFIT/GLM/ILAL/94S-15,http://www.dtic.mil/dtic/tr/fulltext/u2/a285272.pdf, 1994
Nichols, David, “Convert a RIAC 217 Plus Reliability Prediction Calendar Hour-Based Failure Rate to an Operating Hour-Based Failure Rate,” Journal of the Reliability Information Analysis Center, 2nd Quarter 2009
ReliaSoft, “Limitations of the Exponential Distribution for Reliability Analysis,”http://www.reliasoft.com/newsletter/4q2001/exponential.htm, 4th quarter 2001
Schenkelberg, Fred, “The Constant Failure Rate Myth,” http://nomtbf.com/2015/09/the-constant-failure-rate-myth/, Sept. 2015
Leave a Reply