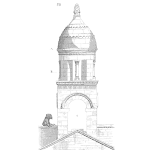
(This is chapter 5 of User Manual for Credible Reliability Prediction – Field Reliability (google.com), cleaned up and typeset for AccendoReliaiblity Weekly Update.)
The nonparametric maximum likelihood estimator for an M/G/∞ self-service time distribution function G(t) extends to nonstationary, time-dependent, Poisson arrival process M(t)/G/∞ systems, under a condition. A linearly increasing Poisson rate function satisfies the condition. The estimator of 1-G(t) is a reliability function estimate, from population ships and returns data required by generally accepted accounting principles.
Background on Reliability Estimation Without Time-to-Failure Data
Reference [1] provides the nonparametric maximum likelihood estimate (npmle) of a reliability functions from stationary M/G/∞ system population ships and returns data. Ships denote sales, shipments mir installed base of a product or parts: monthly, quarterly or annually. Returns denote failures, repairs, replacements, spares sales or returns of parts, for any reason. Except for high cost repairable equipment, there is no incentive to track when returns were shipped. Thus there is no need for time-to-failure or time-to-return data from which to estimate a reliability function.
L. A. Baxter derived a reliability function estimator from ships and renewals data, when all the ships occur at one time [2]. Unfortunately the usual situation is—every month new shipments are made, and returns come back without a clue about how old they are.
Periodic ships and returns counts provide statistically sufficient data to estimate reliability functions. A reliability function is the complement of the service-time distribution function of a M/G/∞ self-service system because ships “serve” for a random time.
Practically every application violates the stationary arrivals condition to represent returns as output from a stationary self-service system. Ships are seldom a stationary process. There are alternative methods to handle nonstationary shipping rates [3], but the npmle of a reliability functions is desirable for its properties.
Extension to Nonstationary Ships
References [4], by Eick, Massey and Whitt and [7] by Meyer Kotkin, permit the reliability function npmle in reference [1] to be estimated for nonstationary M(t)/G/∞ ships and returns, in some circumstances.
The output from an M/G/ service system is a Poisson process with mean G(t). G(t) is the service time distribution. The Poisson mean rate function is analogous to the failure rate function of a time-to-failure random variable. Grenander [5] and later Marshall and Proschan [6] derived the npmle of a nondecreasing failure rate function. The npmle of λG(t) gives the npmle of G(t), because, under reasonable conditions, a function of an npmle is also an npmle.
In the context of ships and returns, G(t) is the time-to-return distribution function. Use its npmle for parts that are returned, whether or not the products continue to function with replacements. Also use its npmle for renewal processes when the probability of multiple failures is small.
The npmle of G(t) can be used when ships are assumed to come from a nonstationary Poisson process, because it’s impossible to disprove for periodic ships: “The Ease of Fitting but Futility of Testing a Nonstationary Poisson Processes From One Sample Path,” [8]. Theorem 1 of [4] says, “The departure process (of an M(t)/G/∞ service system) is a Poisson process with time dependent rate function ?(t) = E[λ(t–S)].” Random variable S represents service time with distribution G(s). If E[λ(t–S)] is nondecreasing, then the npmle of a nondecreasing failure rate function [5 and 6] may be used to estimate E[λ(t–S)] and G(t).
Why not check whether E[λ(t-S)] = ∫ λ(t–u)dG(u) = λ(0)G(t)–λ(t)G(0) is nondecreasing? The integral is from zero to t, because the integrands are undefined or zero for negative values. Plug in the npmle for G(t) and see.
An alternative condition for nondecreasing E[λ(t-S)] is that its first derivative be nonnegative. That is, ? ∫ λ(t–u)dG(u) /?t = ?[λ(0)G(t)–λ(t)G(0)] /?t ≥ 0; the integral is from 0 to t. For example, suppose λ(t) is a linearly increasing function, λo+λt, for t ≥ 0. Then, E[λ(t–S)] if λ(0)g(t) + λG(0) is nonnegative for all t, then the maximum likelihood estimate of E[λ(t–S)] gives a maximum likelihood estimate for G(t).

Table 1. Test condition for npmle estimates assuming linearly increasing ships. (R-sq = 46.74%). The Not1 Test g(t) npmle passes the condition, because all terms are nonnegative. The KISS test passes because the terms are increasing in t.
The npmle of E[λ(t–S)] puts mass at a subset of M(t)/G/∞ departure times (return times in the reliability context). Those masses may be solved for the discrete npmle of the probability density function of G(t). The npmle of E[λ(t-S)] with linearly increasing λ(t) is the npmle of λo +λ[G(t) – G(0) – SUM[ug(u); u=0,1,2,…,t]. The sum from 0 to t runs over the set of return times, u, at which the npmle of E[λ(t–S)] puts mass. This discrete function can be solved for G(t) at jumps. The npmle of G(t) is constant elsewhere.
Suppose the first return occurs at time t(1) > 0 and the npmle puts mass a(t(1)) at t(1). Then the npmle of G(0) is 0 and the maximum likelihood estimator of g(t(1)) is (a(t(1))–λo)/(λ(1–t(1))) if nonnegative. Remaining values of g(u) may be solved recursively for values of u where the npmle puts mass.
The M(t)/G/∞ service system provides a useful model periodic ships and returns for reliability estimation. Information from the M(t)/G/∞ output dwindles as time approaches MTBF [7]. Fortunately many part’s lives are long, even though systems built from them may fail more frequently.
References
[1] L. L. George and A. Agrawal, “Estimation of a Hidden Service Distribution of an M/G/∞ Service System,” Naval Research Logistics Quarterly, 20, 549-555, (1973), https://doi.org/10.1002%2Fnav.3800200314.
[2] L. A. Baxter, “Estimation from Quasi Life Tables,” Biometrika, (1994), volume 81 issue 3 pp. 567 to 577, https://doi.org/10.1093%2Fbiomet%2F81.3.567.
[3] L. L. George, “Estimate Reliability Functions Without Life Data,” ASQ Reliability Review, 13, 21-25, (1993).
[4] S. G. Eick, W. A. Massey and W. Whitt, “The Physics of the Mt/G/∞ Queue,” Ops. Res. , 41, 731-742, (1993), https://doi.org/10.1287%2Fopre.41.4.731.
[5] U. Grenander, “On the Theory of Mortality Measurement,” Skandinavisk Aktuarietidskrift, 39, parts I and II, 70-96 and 125-153 (1956), https://doi.org/10.1080%2F03461238.1956.10414944.
[6] Albert W. Marshall and Frank Proschan, “Maximum likelihood estimation for distributions with monotone failure rate,” Annals of Math. Statist., 36, 69-77, (1965), https://doi.org/10.1214%2Faoms%2F1177700271.
[7] Meyer Kotkin, “The Output of M(t)/G(t)/∞ Queues,” AMSAA, AD-A 120545, July 1982
[8] Barry L. Nelson and Lawrence M. Leemis, “The Ease of Fitting but Futility of Testing a Nonstationary Poisson Processes From One Sample Path,” Proceedings of the 2020 Winter Simulation Conference, IEEE.
Nice work Fred, for translating all those Greek letters into this version of the article.
However, there were a few exceptions in the “Extension…” section of the article.
M/G/infinity
lambdaG(t), and
? ∫ λ(t–u)dG(u) /?t = ?[λ(0)G(t)–λ(t)G(0)] /?t
The “?” should have been the partial derivative symbol instead of “d” in
d ∫ λ(t–u)dG(u) /dt = d[λ(0)G(t)–λ(t)G(0)] /dt.
I will try to clean up my articles so they render better in your Weekly Update