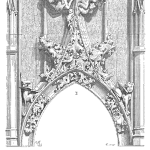
Introducing confidence boundaries
Confidence boundaries can be confusing to reliability engineering practitioners and their audience. Yet, they can play an important role in the risk-based decision-making process. When building statistical models, there is always uncertainty around the model because it is usually based on a smaller sample of the studied population. The confidence interval is the range of values you expect your model to fall between a certain percentage of the time if you run your experiment again or re-sample the population similarly. For example, using a 90% confidence boundary, one would expect 90% of the records to fall between the upper and lower confidence boundaries. As a rule of thumb, the more data you have, the more precise the model and the narrower the confidence boundaries. In essence, if we have an infinite amount of data, we will end up with a perfect model. However, this is never the case. Confidence boundaries help establish the accuracy of the model and also provide some information on the validity of the data.
Example of confidence boundaries
As an example, 2 data sets from the same population are provided below. The time to failure best fit model (blue line) is established using the Rank Regression method. Also plotted are the 90% confidence upper and lower boundaries (red lines). The 90% boundary implies that 90% of the records should fall between both boundaries. It is therefore acceptable that 10% fall outside of those boundaries. The data set on the left (Model 1) is in the early stages of the operation where only 3 records are available. Later on, with more records, we end up with the graph on the right (Model 2). The models evolve over time; the Weibull distribution parameters beta and eta change. Model 2 is still acceptable even if one point falls out of the confidence boundary. This is because “90% within the boundary” rule is still maintained.
The graph on the left (Model 1) with the lowest number of points, has the wider confidence boundaries. With more points, Model 2 is deemed more precise and the information it will provide will be more accurate. Therefore, it has narrower confidence boundaries. However sometimes we do not have a choice when dealing with records; we end up with very little data and have to make the best decision.
Using Confidence Boundaries for conservative Risk decisions
Let us assume that the operator has to make a decision based on very small number of records; i.e., 3 as in Model 1. That decision could be something like; what is the unreliability or the probability that the equipment fails at or before 10 days following a repair? Using the Model 1 probability plot above, we obtain the following:
- The best fit model (blue line) tells us that the probability of failure (F) at or before 10 days is 18%. Conversely, Reliability at 1-F equals 82%.
- The most conversative or less optimistic value based on the lower confidence value is 44%. Conversely Reliability at 1-F equals 56% – see above graph.
- The least conversative or more optimistic value based on the upper confidence value is 0%. The upper confidence boundary does not intersect the 10-day value on the time axis so this implies that the asset cannot fail at or before the 10-day mark.
Being overly optimistic is not a recommended strategy in risk management. Even though we do “hope” for the best, we have to “plan for the worst” case. However, by worst-case, we mean the worst “realistic” case rather than the “world is falling apart” case. Therefore, knowing that the 3-point statistical model is not optimal yet having no alternative, a prudent operator would lean toward the lower confidence boundary. In this case, the probability of failure at or before 10 days of would be 48%. Risk is a product of probability and consequence so the risk-based decision will be made using this value.
Looking at Model 2 when we have more data, the probability of failure at or before 10 days is will have increased to 25% for the best fit model but reduced to 42% for the lower confidence boundary. Those values are not very far from the Model 1 values so in essence Model 1 was not so inaccurate after all. Note that this similarity is based on this data set only and should not be taken as a general case.
In reliability engineering terms, “a little data is better than no data at all”. As shown in this example, using the limited amount of data we have, and using confidence boundaries, it is possible to define a conservative approach to risk management.
Thanks Andre, great analysis. for most applications and testing, I usually take the more conservative approach by recommending the lower boundary values when performing failure data analysis.
Question?
In application, How would you differentiate between the single and double sided confidence level?
Thanks for your question Damilola
I am not sure I understood your question. A few thoughts….
You could use either (single or double) depending on your needs.
I prefer using double confidence boundaries for two reasons:
1) I can see if I have a good fit for my data. I.E. how the points fit in the confidence boundaries
2) for the reasons highlighted in the article – if a customer wants to get a conservative risk approach, I would use the low confidence boundary.
I hope this makes sense otherwise feel free to ask.
Andre