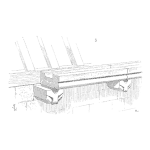
Over the past week, I received a couple of interesting questions. One concerned assuming a Weibull beta value for an accelerated life test plan. The second involved assuming expected life models for elements within a reliability block diagram.
In both cases, we faced incomplete data and uncertainties, yet felt the need to assume some values in order for the math to work out. We do make assumptions in order to solve problems. We also can make mistakes that lead to unwanted consequences.
For example, if a mechanical engineer is designing a load-bearing beam and assumes the material strength is larger than it is in reality, the beam will collapse. In this simple example, the assumption was the mistake.
Sometimes, the assumptions we make are fine and sometimes they mislead us and may lead to mistakes. So, how can we know when an assumption is reasonable or when it will lead to problems?
Why and when do we make assumptions?
As engineers, we are tasked with solving problems. Given the complexity of even a simple component, we use assumptions to simplify the problem allowing solutions. Our tools and understanding allow us to avoid overly simplifying assumptions, yet even simple problems often require some use of assumptions in order to solve the problem.
For example, back to the beam example, before we understand the variability of manufacturing beams, we may have assumed there was a minimum strength for a specific type and size of the beam. Later, with information about the variability of finished beam strength, we could use the distributions of strength and expected loads to conduct a statistical-based strength – stress analysis. Yet, we are aware that no beam is homogeneous, that upon closer examination we may be able to identify stronger and weaker portions of the beam, yet we often assume the material to of uniform strength.
In reliability engineering, we once assumed all failure rates occurred with a constant hazard rate. Today, we know (at least you should know) that very few actual failure mechanisms occur that are well described using a constant hazard rate. Today, we have the tools to calculate system models (block diagrams, fault trees, etc.) using the suitable time to failure models for each element within the model.
Yet, within many types of analysis, we assume each element performs over time independently of other elements within the system or between systems. This is also rarely true, yet we have found the added complexity to model the interactions and dependencies complicates the solutions unnecessarily. It costs more to add more detail.
The difficult part is solving problems with the right amount of assumptions such that we can solve the problem in a cost-effective manner and have a high chance of finding the right (it works as expected, is what I mean here) solutions.
How and why do mistakes happen?
In every project I’ve ever been a part of, ‘mistakes have been made’. We build prototypes, conduct experiments, analyze and evaluate our decisions in order to identify the mistakes and correct them. If our project involved designing a load-bearing beam and upon loading the beam it failed, we have the opportunity to correct the mistake, possibly concerning the assumed strength of the beam.
Some mistakes are willful. We decide to take a shortcut to save time or money with the basic faulty assumption that it will be ok or no one will find out. Have you ever agreed to substitute one vendor’s component for another one, when assuming since it was the ‘same form, fit, and function’ we would not need to check that assumption? As many of you know, this is not always a good assumption to make, yet it does work in many situations.
Another way mistakes occur in engineering is the lack of time, resources, and understanding. Yet, even when given ample time and resources to check each and every design decision, we are certain to not find all mistakes. There are too many decisions, too many assumptions, and too little time.
Yet another way we make mistakes is out of ignorance. Let’s say during your course work and studies and to date, the same formula to size a beam has worked without any problems occurring. Yet, let’s say there is a material processing change that increases the resulting range of beam strengths. Some percentage of beams now are significantly weaker unknown to you. Where is the mistake land now? A beam will fail despite the engineer’s knowledge of the change to the materials.
Yet another worth mentioning is mistakes can happen out of willful ignorance. You may choose to ignore learning a better analysis method that allows you to avoid assuming a constant hazard rate, for example. Another example is assuming your engineering judgment is good enough to avoid having to check your work.
There are other ways mistakes occur, yet you get the idea there are many paths that lead to mistakes and failures.
Sometimes an assumption is a mistake
We have to make assumptions to solve engineering problems. Trusting improper or incorrect assumptions lead to mistakes. That being said the real issue here is understanding the role assumptions play in our work. Consciously identifying our assumptions and understanding the risk that assumption poses is also part of our work as engineers.
If assuming a life model for ALT planning or system reliability modeling, what is the consequence of being wrong just a little, or a lot? Will the results change the decision to trust the beam just designed?
A good practice is to identify the salient assumptions in your work, including those being made behind the ‘screen’ in your analysis software packages, btw. Document the assumptions. Show your work.
Second, for the assumptions that have an uncertain impact on the results or are based on a poor understanding of the impact, plan to get more information. Test your assumptions. If assuming the data is normally distributed does that matter for your analysis or not, and if it does matter, take the time to determine the appropriate distribution and alter the analysis to accommodate the non-normality.
Finally, accept we will make poor assumptions. We will make mistakes. Learn from them. Share your knowledge. Then make better assumptions and fewer mistakes.
Why assume? Test assumptions!
There are tests for Weibull shape parameter, (even without life data! https://sites.google.com/site/fieldreliability/home/why-kill-controls/):
Ho: shape parameter accelerated is same as unaccelerated vs.
Ha: shape parameter accelerated differs from unaccelerated
HALT proponents may object, but I distinguish between ALT and HALT life tests as: HALT changes the distribution function of life in addition to changing lifetime mean, median, or other location parameter and ALT doesn’t.