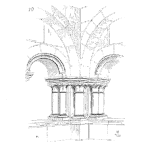
Ensuring Reliability Data Analysis Leads to Positive Action
Convince, don’t confuse! Justify, don’t exaggerate!
Project managers want to deliver their product on time and on schedule. Design engineers want to believe that they have got it right. But your analysis, test results and field data suggest that there might be a problem. What do you do?
The key words here are “suggest” and “might be”. How should you present your evidence and analysis such that it doesn’t exaggerate with certainty, or confuse with statistics? How should you ensure that your conclusions lead to positive action?
Reliability Data Analysis is a Powerful Tool
The Big Issue Requires Immediate Action
Poor field data? Don’t have operating hours, only days-since-installation? Can’t link returned items to field location? Can’t trust repair action reports?
Many of us will have experienced corrupt and incomplete data, and yet this is the data we have and we need to do our best to analyze it.
And what if the outcome is early indication of a BIG issue?
If you wait until you have unassailable evidence, the issue will have become BIGGER. If you stand up and present your evidence early, you had better be ready for challenges. You know the holes in your data and analysis, so you certainly can’t exaggerate and claim certainty. But if you hide behind statistical confidence intervals, you’ll likely be ignored.
So, how should you present your conclusions and justification?
Remember that design engineers and project managers are also smart people
Design engineers and project managers are used to undertaking analysis and interpreting charts and diagrams. They just probably aren’t always expert in interpreting Weibull graphs and statistical confidence intervals. Add uncertainty from having incomplete and questionable data, and you have a recipe for not being believed.
My experience is that it is best to be honest about the sources of uncertainty in your analysis, but also to set boundaries on the uncertainty and to give the nature and effect of each uncertainty. Guide your audience through how you reached your conclusions. My experience is that when your audience feels a part of the analysis, they are more likely to buy-in to its conclusions.
Of course, there is the risk that they don’t agree. So, it is always good to prepare yourself by thinking up potential counter-arguments, and preparing your responses.
Acknowledge the gap in data, but turn it to advantage
But not all failures have been analyzed and repaired. We don’t have sufficient numbers. We need to wait until we get more recent failures back to the repair centre.
Can you establish the delay-time for getting failed units to the repair centre, and show the effect of waiting on the magnitude of the issue? Use it as an argument to initiate special action to get units back from the field.
Pre-empt the counter-argument
But our warranty claims for this issue are only 1% of products shipped. There is always variation in claim rates, month by month.
Show the difference between average claim rates over the warranty period, and age-related failure. Show how averages hide age-related trends until it is too late.
Be thorough, but keep it simple
How do we know that the issue that is highlighted is real, and not some phantom spike in the data?
You know that this possibility exists, so you can’t deny it. But you could highlight the period over which the issue has been present. You could highlight the number of events that are being considered. Use colour to enhance your graphs, to attract attention to your major points. Include confidence intervals, but keep it simple. Take them through the analysis, showing how you assessed the level of uncertainty, and how you concluded that the issue or trend needs action. A good rule is to ask, “Could my teenage son or daughter understand this?”
Case Study: Early Reliability Data Analysis Enabled Pre-emptive Action
I was asked to analyze some data shortly before a Company holiday. A fellow engineer had noticed several product failures that hadn’t been seen before. He asked me for help with life-data analysis. Together, we reviewed the data pool for signs that the issue came from particular customers or from particular manufacturing batches. We checked for any obvious source of data corruption or limitation.
Be open with regard to data limitations and show what you have done to minimize their impact.
The major limitation in the data was that product-operating hours were not available. However, the product is generally switched on 24 hours per day and so this was not an issue. The module in question had multiple installations per product, and the installed position of any particular module and its repair is not known. Therefore, we chose to include only first failures in our analysis. As it turned out, this did not reduce our data pool; we did not then have any 2nd failures.
When the data was analyzed using Weibull, we found that the rate of failure was increasing with a beta>>1, even though only a very small percentage had failed at that time. Confidence interval on beta did not include a beta<1, and therefore we could declare that the rate of product failure was very likely going to increase.
Highlight the important basis for your concern
Our engineering team had previously received a lunch-time overview of how to interpret Weibull graphs, and so we were able to show the Weibull analysis graphs directly. Our software was able to declare “goodness of fit” measures to confirm that Weibull was the best fit, but the main presentation was the single Weibull line and 90% confidence interval lines. However, the key take-away we highligted from this was that we could expect x% failures by 2-year life and y% by 5-year life (the customer expected life)
Translate Weibull statistics into easily understood take-away numbers.
We then translated this into future customer returns month-by-month, based on the customer product shipments.
Translate analysis into other commonly understood metrics
Outcome
In summary, we needed to deliver a call to action to address what we believed was a major issue.
We had declared that not all failed units had been returned to the repair centre:
We recommended that direct action be taken to get remaining units returned and to initiate a call-back for all future failures of this type.
We needed to get more evidence
We recommended an immediate root-cause analysis of the failures, by a cross-functional team.
We needed to undertake more analysis
We undertook to regularly update the field data analysis.
The Reliability Team were involved.
We were key members of the ongoing analysis. We were part of the solution.
The outcome was that the Company was able to achieve early containment, was able to develop a work-around, was able to update their design methods, and was able to update their lessons-learned.
There has been a $$-cost to the Company, but early action has prevented adverse customer impact.
Conclusion
If we, as reliability engineers, don’t present analysis in a form that engenders belief and buy-in, then are wasting our time. We have to deal with real-world issues, often with imperfect data. If we claim to be certain when we shouldn’t be, our colleagues will learn to mistrust us. If we hide behind uncertainty, and don’t interpret to show likely outcomes or a conclusion that allows for the uncertainty, then we are requiring people less expert in our field to take responsibility we are unwilling to take. They will not respect us.
The middle ground is to acknowledge and explain the sources of uncertainty, to show what you’ve done to minimize it, and to highlight the conclusions you can still make, notwithstanding the uncertainty. When presenting your analysis, highlight the basis for your concern, highlight key take-away points, and translate your analysis into commonly used metrics. Recommend action, and be part of the solution.
In this way, you will generate trust and respect, and you will be effective in achieving product reliability.
Please share your stories. If you would like to comment on this article or pass on your experience, please contact me via the Contact Form below. For further information, visit my Contact Page, or visit my website www.lwcreliability.com.
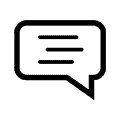
I enjoyed reading your article, especially the Case Study. What did your RCA of the failures show?
Was it a design deficiency or manufacturing/assembly defects?
Hi Spiros,
The RCA found fatigue failure of solder joints due to thermal fatigue. This had been considered during design analysis and modelled results had looked good. However, the modelling detail to account for material property variability had been imperfectly “keyed in”. A lesson was learned. It was a design weakness.