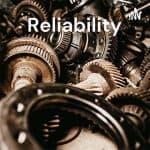
Statistics for Reliability Engineers Part II
In this episode, I speak with Luke De Jager and hear his thoughts on the level of Statistical knowledge required to become a good Reliability Engineer. This is a very open-ended question as the logical answer to this problem seems to be, it depends. The dependencies arise from what you want to achieve as a Reliability Engineer.
Listen to this podcast, if you want to learn more about the depth of Statistics required for a Reliability Engineer.
Listen to this podcast, if you want to learn more about the depth of Statistics required for a Reliability Engineer.
If you have any questions for Luke, you can contact him on LinkedIn.
Let me know what you think of this episode. Please share your feedback with me!
Interesting podcast. Thank you Fred
I very much enjoyed the podcast.
Speaking as a statistician, statistics is important when it is necessary to convert data into actionable information. Sometimes, this is simple, and sometimes it is complicated. Often, there is a need to combine knowledge of physics/chemistry to failure with available data, implying that many challenging reliability applications require collaborative teams.
Luke’s discussion of the need for special methods (beyond common “Weibull analysis”) for repairable systems data (more generally known as recurrent events data) was spot on.
For some kinds of reliability applications (not all), we are in the era of “big data” (because of modern sensor and communications technology), and the potential for the use of machine learning techniques in reliability is huge. However, the generic machine learning techniques work well only in applications that are primarily interpolative.
Linking in physics of failure in these kinds of applications is a current area of research.
Finally, back to statistics, one of the most important contributions of statistical methods is the quantification of statistical uncertainty (i.e., uncertainty due to the limited information in the available data). Some of the most challenging applications I have been involved in have been where there is “big data” but “little information.” In one application, there were millions of units in the field but only a handful of failures—with the question of how many more failures can be expected in the next ten years.