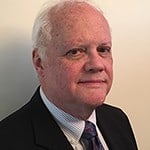
Introduction
In the prior article, Process Capability IV, vehicle wheel toe alignment showed excessive variation. Because the vehicle assembly process is very long and involves many steps, the toe alignment problem required brainstorm which serial production steps, factors, and levels could be responsible for the variation. The most likely process steps were examined and the problem area identified as a wheel alignment machine that needed maintenance and calibration.
Sometimes a production process has a very short production sequence. A single machine or process step may be responsible for excessive variation. While it is generally possible to center the process, it is harder to reduce process variation. A systematic method of examining multiple factors is presented here.
Historical Approach
A common approach to process improvement is changing one-factor–at-a-time (OFAT). A factor would be selected and it’s setting adjusted to a different value. The output would be monitored, hoping for improvement. If the response improves, then a decision needs to be made? Stay with the new setting or adjust again. Alternatively, if the response degrades, then the factor would be adjusted to another value, again hoping for improvement.
This approach relies on personal experience and people have different experiences. Frequently there is disagreement as to which factor to adjust and what setting to use. Using this method is very slow and time consuming. The OFAT method ignores the potential for interactions among factors.
Design of Experiments (DOE)
When there is a production problem, like excessive process variability, production is not shut down unless it results in a safety issue, non-functional parts, or visible defects. However, manufacturing personnel will try to adjust the process to improve capability by adjusting process factors. Many parts are going to be produced at each setting or combination of settings. Rather than the OFAT approach, the DOE approach is more efficient.
DOE provides systematic ways to examine multiple factors to determine their effects and interactions on a response variable. During the analysis, the standard deviation is estimated and the statistical significance of factor effects estimated. If the response is a dimension or a force that can be quickly measured, then test results and the analysis can be accomplished quickly. If the response is a production defect rate, then a significant volume of parts need to be produced to determine the defect rates at different factor settings.
Again the process starts with brainstorming potential factors responsible for the problem and organizing the results with the fishbone diagram. There will probably be too many factors to examine in one DOE. A good practice is to plan for 3 test stages. Budget about a third of the cost and time to each DOE. If management wants a cost or time estimate, the total cost and timing plan can be reported.
For the first DOE, the team selects factors to adjust and the level settings for each factor. The next set of experiments will be planned after results from the first DOE are analyzed. Assuming important factors have been selected, the first DOE will identify statistically significant factors and the direction to adjust factors for process improvement.
Case Study – Casting Voids
An Aluminum casting plant needed to produce a manifold for an internal combustion engine. The manifold guides inlet air to individual cylinders or combustion exhaust products from the cylinders. The design is determined by engineering, but the casting process is under plant control.
The casting process used foam form packed in a sand matrix. In the foundry, a major defect was casting voids. During initial production, it was common to have high defect rates on the order of 30 to 50%. After a few years of using OFAT methods, it was common to achieve 2 to 5% defects. The plant management decided to try DOE methods to reduce casting voids.
The factors that affected void defects were brainstormed. Some of the high level factors included the molten aluminum temperature, the pour rate, and the quantity. Others included the orientation of the foam form in the sand and the location of vent and metal holes. Even the type of sand, the particle size, and tamping force were considered. Generally, each factor was set to two different levels. For example, there could be two different molten Aluminum temperatures, a high and a low pour rate, …
If 7 factors and all of their possible interactions are analyzed, a full factorial DOE requires 128 tests, assuming 2 levels for each factor. A screening DOE could be used to examine the 7 factors with 8 tests. The metric was defects per 100 manifolds. At each factor-level, 100 manifolds were cast, so 800 manifolds were required for the screening experiment. While this sounds like a lot, a typical manufacturing process could produce this volume of parts in a day. If one used OFAT methods, then they probably would produce hundreds of parts at arbitrary settings without making significant improvements.
The results of the first DOE were process settings that reduced the void defect rate to the 2 to 5% level, not normally seen in initial production. Subsequent DOEs reduced the void defects to levels that were not detectible with 100 parts at each factor-level combination. The sample size needed to be increased to about a 1000 at each factor level combination.
Conclusion
It improve process capability, the DOE method
- Can be employed where manufacturing can continue where processes are not capable.
- Is faster and less expensive than OFAT methods.
- Provides information on which factors are significant for the process.
If anybody wants to engage me on this or other topics, please contact me. I have worked in Quality, Reliability, Applied Statistics, and Data Analytics over 30 years in design engineering and manufacturing. I taught undergraduate statistics; reliability and robust design at the graduate level. I provide Minitab seminars to corporate clients, write articles, and have presented and written papers at SAE, ISSAT, and ASQ. I can help you solve manufacturing and design problems.
Dennis Craggs, Consultant
810-964-1529
dlcraggs@me.com
Leave a Reply