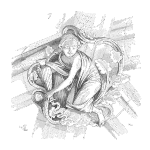
One of the most often asked questions of reliability professionals (or statisticians) is related to how many samples for a test? This typically is not an easy question to resolve without some work and knowledge of the testing in question. We are often asked anyway, and expected to have an answer.
While not the most often asked question on the CRE exam, you might see something related. Budget planning, prototype counts, test equipment sizing, etc. all need an estimate for sample size.
While the best sample size calculation is to calculate it after the test is completed, that really isn’t a practical approach. So, how to get started? The idea that a test item can either pass or fail the test permits us to treat each samples experiencing the test as a Bernoulli trial. Thus, we can use the binomial distribution to determine sample sizes. With a little experimentation with the binomial formulas, one can find the minimum number of samples is always associated with a test design expecting (and permitting) no failures.
So, if we run n samples for one lifetime (might be accelerated) of use, we can demonstrate with C confidence at least an R reliability.
$$ \large\displaystyle n=\frac{\ln \left( 1-C \right)}{\ln \left( R \right)}$$
The derivation is in Wasserman’s book Reliability Verification, Testing, and Analysis in Engineering Design.
We do not need to make an assumption about the life distribution, just have to subject each test unit to the same stress that represents one lifetime of use. For example, if a car door is expected to operate 10,000 times in its life, then using a robot to open and close the door 10k times would represent one lifetime. Note: one should carefully consider other contributing stresses and the specific failure mechanisms, such as temperature and contamination as it may be as important as the number of cycles.
The other assumption is that each test item has the same chance of failing or passing the test. The items are the same or within manufacturing expected variation and they respond individually to the applied stresses and measurements.
C or confidence is the probability that the sample will provide results consistent with the actual (unknown) performance. This is similar to the producer’s risk or the 1-α type of confidence. In practice, the lower limit for C is 0.6 or 60%. Consider that at 50% the test results are 50/50 likely to represent the population. Better or worse reliability is not clear. Lower than 50% confidence even further reduces the odds of a meaningful result.
R or reliability is the probability that the population will survive for one lifetime. Or, given 100 units, how many are still working in one lifetime.
For the sample size calculation, we are often given reliability either by system or subsystem goals. And, we often have local policies concerning sample risk (confidence). Thus, one can quickly determine the number of samples required for the test using the above formula.
So, as a quick example, that I can remember and use often. If we want to show the new product has at least 90% reliability with 90% confidence, we need 22 (round up) samples to experience one lifetime of stress without failure.
If there is any failure, then this formula does not apply, and one cannot conclude the product has 90% reliability with 90% confidence. Altering the confidence after a failure is not a good practice (my data analysis course professor called it evil). If there is a failure, one technique to salvage meaningful information from the test is to continue to run till there are at least five failures. Then fit an appropriate life distribution to the data. This is a risk of the success testing approach.
The success formula for sample size is each to remember and calculate. It provides a reasonable way to consider the number of samples needed to demonstrate reliability. In another post, we’ll consider methods to reduce the sample size requirements further – yet these often involve more restrictive assumptions or unique situation.
For now, consider adding this formula to your short list for regular use.
Related:
Success Testing Formula Derivation (article)
Hypothesis Test Sample Size (article)
Extended bogey testing (article)
I have an automotive part durability test for 10K cycles with 90% C and 90% R which would require 22 samples using n= ln (1-C)/ln (R). I’m trying to reduce the number of samples by increasing the durability cycles. Is there a calculation to work this out?
Hi Ernest, add m (number of lifetimes) in front of the ln(R)
If 10k cycles represent the expected one lifetime of use, then m = 1 thus drops out of the equation. If you run 20k cycles to twice the expected lifetime number of cycles, then m = 2 and cuts sample size, n, in half.
Beware that as you increase the cycle count you may incur failure mechanisms that are not relevant to expect lifetime use – so be cautious when increasing m. For example, if there is an acceptable wear out mechanisms that occurs at around 12k cycles, with a very low probability of occurring over 10k cycles, then you would likely have failures when trying to achieve 20k cycles, m=2 and the test would fail to demonstrate the 90/90 desired.
cheers,
Fred