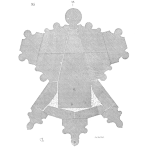
Favorite Questions and Answers from my Course “Statistical Process Control (SPC) Using Microsoft Excel”, Pt 2
In this second installment of my three-part article series, I am again showcasing some of my favorite student questions from the past seven years since first launching my online class titled “Statistical Process Control (SPC) Using Microsoft Excel.”
Each exchange represents not only the question in that student’s mind, but the type of question that lingers the minds of countless professionals trying to advance their skillsets. Each exchange – some of which I edited for clarity – become a permanent part of the class itself that future students can read and learn from. Perhaps you’ve had some of these questions as well.
Question:
Ray,
My process reads above 2 Sigma level, a few points and below 3 Sigma line, my question is our customers are not concern and never asks us why is that. Should I be concern? Why are points above the 2 or 3 sigma line is a big deal?
Now these sigma levels on the control chart are statistical levels and my customer spec are wider than control limits. My customer seems happy … never complains. Should I go back and tighten my process and why should I do that? If I try to make my process in control or below the 1 sigma line, I may mess things up!!
Thank you
Adam
Answer:
Hi Adam,
Thanks for taking my SPC class … It sounds like this class is making you think about the charts you’re producing.
I think I understand your scenario, and if I’m picturing this correctly, the spread of your data is pretty narrow, but the whole thing is shifted 2 to 3 sigmas away from the nominal.
On one hand, if you’re customer is happy, then you should be fine. The problem is that one day, your customer may stop being happy. Over and over again, I’ve seen where a new person steps into an old situation, and suddenly wants to see some improvements.
Plus, distance between your process limit and your spec limit is like a little insurance policy against unforeseen shifts in your process that might, in your case, result in defective product. A process closer to the center of the spec range protects your organization against these sudden shifts.
Another thought related to Taguchi’s Loss Function is that when your process is shifted away from the target, someone is paying the price for it. I don’t know your process, but if this 2-to-3 sigma shifts is the result of extra material being added to a feature, then maybe shifting your process closer to the target would be a cost savings for you.
I hope some of this may be a help to you. In the end, I would wonder, if in fact your customer is largely unconcerned about your capability, then why are you collecting and analyzing all this data? It feels like a futile exercise.
Take care, and let me know if I can be of any more assistance to you.
Question:
“Hello,
I have a question regarding the sample size. Please explain how do we select the sample size for different population sizes.
Thanks in advance,
L”
Answer:
Hello L … Thank you for taking my SPC class. That’s an excellent question, and one I’ve received many times. There’s a short answer and a long answer to that question.
The short answer, and the practical one, to selecting a sample size for your control chart is to consider the measurement system and how long it takes to perform the measurements relative to the rest of the work the operator is doing. Then pick one of the popular sample sizes, often an odd number, like 3, 5 or 7. The statisticians of Shewhart’s day carefully developed the Table of Control Chart Constants to adjust for the various sample sizes.
The long answer involves establishing alpha and beta risks, and inputting them into elaborate statistical equations. As a guideline, more samples are better if you want to minimize the risk of error, but it comes at the cost of measuring them.
Google “sample size formulas” for more details.
Question:
“Hi Ray, I have a question about the sample data. Rather than sample data, does it make sense if we will take actual full data? The UCL & LCL and range calculation will be the same?”
Answer:
Hello Sheau,
So good hearing from you. I hope you’re enjoying the SPC class so far.
The goal of SPC, and all of inferential statistics, is to characterize the population based on information you’ve gained about a sample. In manufacturing, this is quite useful because the populations (every part produced) are usually quite large, making the measurement of each part quite expensive.
But if your production volume is low where you can measure every part, then yes, that is better than SPC.
I hope that helps. And thank you again for reaching out.
Question:
Dear Sir,
My question is related to the U chart.
We are using the u chart at in-process and final inspection stages, and every day we inspect PC boards with different batch sizes and monitor the defects.
The data is collected monthly, and new control limits are calculated with previous month data i.e. we plot March’s data on a U-chart with control limits calculated from February’s data.
Is the method followed is ok. Kindly clarify.
Regard, Jithesh
Answer:
Hello Jithesh,
So good to hear from you. I am glad to hear that you’re applying the methods you learned in SPC to your workplace.
The only concern I have is that you’re re-calculating control limits every month. That should not be necessary.
Re-calculating control limits is necessary when there is a process change of some sort .. raw material, tooling, process settings, etc. And verifying your control limits is a good idea. But as a general rule, once the control limits are established, there is no need to keep changing them. They represent the normal range of the process. Unless your process changes, then your control limits shouldn’t change either.
If you are finding that your control limits change significantly from month to month, then your process is out of control and you should investigate the reasons why that is. Or perhaps you didn’t capture the full process variation when you first calculated them.
I hope that helps. And thank you again for taking my SPC class. I sincerely hope it continues to provide answers to your manufacturing problems.
Ray Harkins is the General Manager of Lexington Technologies in Lexington, North Carolina. He earned his Master of Science from Rochester Institute of Technology and his Master of Business Administration from Youngstown State University. He also teaches manufacturing and business-related skills such as Quality Engineering Statistics, Reliability Engineering Statistics, Failure Modes and Effects Analysis (FMEA), and Root Cause Analysis and the 8D Corrective Action Process through the online learning platform, Udemy. He can be reached via LinkedIn at linkedin.com/in/ray-harkins or by email at the.mfg.acad@gmail.com.
Leave a Reply