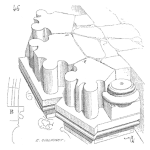
In hypothesis testing, we set a null and alternative hypothesis. We are seeking evidence that the alternative hypothesis is true given the sample data. By using a sample from a population and not measuring every item in the population, we need to consider a couple of unwanted outcomes. Statisticians have named these unwanted results Type I and Type II Errors.
A Two-Way Decision Process
Unknown to us, hence wanting to conduct a hypothesis test to learn something, the null hypothesis for the population under study may actually be true. On the other hand, the null hypothesis may not be true.
The view of the population we have is via the sample taken. We will make a decision based on the sample and determine if we accept or reject the null hypothesis.
This set of four possible outcomes is often presented as a two-way decision process as so:
Decision | Null Hypothesis | |
---|---|---|
True | False | |
Reject H0 | Type I Error α | Correct 1-β |
Accept H0 | Correct 1-α | Type II Error β |
When the actual null hypothesis is true and our sample indicates that is the case, that is a reflection of what is correct. We may denote this with $-1-\alpha-$.
When the actual null hypothesis is false and our sample indicates that is the case, that is again a reflection of what is correct. We may denote this with $-1-\beta-$.
When the sample leads to a decision that conflicts with what is true for the population, we have an error.
Type I Error
As you can see in the table above, a Type I Error occurs when we reject the null hypothesis based on the sample when the actual unknown population agrees with the null hypothesis.
We reject the null hypothesis when it is true, thus making an error, even though the sample and calculations indicate that we should reject the null hypothesis. This occurs due to the set in the sample happened to have values that would indicate we should reject the null hypothesis. The collection of the sample has a chance of causing this result or error.
This error is also called the producer’s risk and often indicated by the level of significance denoted by $-\alpha-$. Determine $-\alpha-$ as a risk of incorrectly rejecting the null hypothesis. If we would accept that 1 in 20 times the sample drawn would result in this error, we have a risk of 1 in 20 or 0.05 or 5%.
We often approach this risk by stating what confidence we desire in the sample. A 95% confidence equates a 0.05 level of significance since $-C=\left(1-\alpha\right)100\% -$.
Type II Error
Again referring to the table above, this error is the case when the unknown to us state of the null hypothesis is false. Then based on the sample drawn decide to not reject the null hypothesis. Given the double negative of ‘not reject,’ it usually takes a moment to realize that we are unwittingly convincing ourselves that the null hypothesis is true.
In short, this error occurs when we do not reject the null hypothesis when in fact the population does not agree with the null hypothesis.
We denote a Type II Error with $-\beta-$ and it is often called the consumer’s risk. We set $-\beta-$ much as we set $-\alpha-$
When All is Correct
The other two regions of the two-way decision process described above are when we get the decision right, in other words matching the unknown reality.
The region with the decision to reject the null hypothesis when it is in fact false has the $-1-\beta-$ term, which is called the power. It indicates the probability that the sample will provide sufficient evidence to correctly reject the null hypothesis. This term is interesting and will be the subject of another article.
When preparing to conduct a hypothesis test we often only consider the level of significance or confidence and allow the power to be whatever it turns out to be for the selected sample size. We can set $-\beta-$ yet it is impossible to minimize both $-\alpha-$ and $-\beta-$. The two errors are inversely related. Thus for a fixed sample size increasing one proportional decreases the other.
Leave a Reply