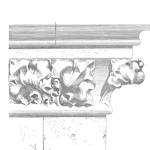
Data-driven decision-making is central to designing and improving products and processes. Professionals are often presented with statistical analyses, with key outputs such as p-values or confidence intervals that indicate whether results are “statistically significant.” However, statistical significance doesn’t always translate into meaningful changes on the shop floor or within a product’s design. Understanding the difference between statistical significance and practical significance is crucial to making well-informed decisions that genuinely impact the business.
Statistical Significance
Statistical significance refers to the likelihood that the observed results are not due to random chance. Typically, this is assessed using a p-value, which indicates the probability of observing the data, or something more extreme, assuming the null hypothesis (i.e., no effect or no difference) is true. When the p-value is below a pre-set threshold (commonly 0.05), the result is considered statistically significant, meaning the null hypothesis is rejected in favor of the alternative hypothesis.
However, statistical significance alone does not convey the magnitude of the effect or whether that effect is meaningful in a practical context. A small difference in a process metric may be statistically significant because of the sample size or the low variability of the process, but it may not justify any real-world action.
Practical Significance
Practical significance, on the other hand, focuses on whether the results have real-world importance. In manufacturing, this could be measured by the effect on product performance, cost reduction, or improvement in reliability. Practical significance is subjective and depends on the context, such as production volume, cost, and customer expectations. A statistically significant result can have no practical significance if the change it reveals is too small to make a difference in practice. For instance, improving a machine’s cycle time by 0.1 seconds may be statistically significant, but if that improvement has no measurable impact on throughput or costs, it is not practically significant.
Example 1: Service Life
In the context of reliability engineering, let’s consider a product’s service life—the period during which the product is expected to perform its intended function without requiring significant repairs. Suppose a reliability engineer conducts life testing on a new mechanical component designed for heavy-duty applications. The engineer compares two different materials used in the component, one of which is more expensive than the other. After analyzing the test data, the engineer finds that the more expensive material statistically extends the service life of the component by 2%.
A hypothesis test yields a p-value of 0.03, indicating statistical significance. However, the engineer must evaluate whether this 2% increase in service life is practically significant. If the original component already had a long service life (e.g., 20 years), a 2% improvement would only add a few months of additional use. For many customers, this small increase might not justify the added cost of the more expensive material, especially if the product’s service life already exceeds their expectations.
In this case, despite the statistically significant difference in service life, the practical significance may be low, and it might not be worth implementing the change.
Example 2: Reducing Scrap Rates
In another scenario, a quality engineer is investigating ways to reduce the scrap rate in an injection molding process. After experimenting with different temperatures and pressures, the engineer finds that changing the mold temperature from 180°C to 185°C reduces the scrap rate from 2.5% to 2.3%. A t-test yields a p-value of 0.01, demonstrating statistical significance.
However, this 0.2% reduction in scrap rates may not be practically significant. If the company produces only a few thousand units per month, the cost savings from reducing scrap by 0.2% may not justify the operational adjustments and the cost of recalibrating the machinery to maintain the higher temperature. On the other hand, if the company is producing millions of units monthly, even a small reduction in scrap rates could translate into significant cost savings.
Bridging the Gap
Understanding the difference between statistical and practical significance is vital for making sound decisions. While statistical significance can alert you to meaningful differences in data, practical significance requires an assessment of the real-world impact of those differences.
One strategy for framing the potential for improvements is to define practical significance at the start of your investigation. Before conducting a study, define what magnitude of change would be meaningful in a business context, considering factors like cost of implementation and customer impact. This framing of practical significance sets the threshold over which a design improvement must pass in order to justify further consideration.
While statistical significance is valuable in determining the validity of a result, it is the practical significance that determines whether a change will have a meaningful impact on the business. By considering both, professionals can make better decisions that lead to tangible benefits in process performance and product reliability.
Ray Harkins is the General Manager of Lexington Technologies in Lexington, North Carolina. He earned his Master of Science from Rochester Institute of Technology and his Master of Business Administration from Youngstown State University. He also teaches manufacturing and business-related skills such as Quality Engineering Statistics, Reliability Engineering Statistics, Failure Modes and Effects Analysis (FMEA), and Root Cause Analysis and the 8D Corrective Action Process through the online learning platform, Udemy. He can be reached via LinkedIn at linkedin.com/in/ray-harkins or by email at the.mfg.acad@gmail.com.
Leave a Reply