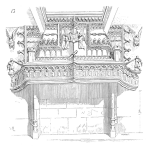
Would you like the reliability of all your products and their service parts, without assumptions, in real environments, and with all premature failures, complaints, repairs, warranty expirations, preventive maintenance, changes, warranty extensions, etc.? Field reliability tells what really happens!
Would you like credible reliability predictions, not just MTBF predictions, using age-specific field reliability? Fred Schenkelberg invited me to show Hewlett-Packard people how, a long time ago. Anybody could do it. Thanks Fred.
Remember actuarial rates? They are discrete, age-specific, nonparametric failure rates: mathematically equivalent to reliability functions. Nonparametric statistics avoid assumptions and preserve all relevant information in data (Walter Shewhart’s rule 1).
Some people say that you need life data to make reliability estimates; e.g., table 1 shows typical grouped life data: ships and returns counts. Column “Ships” lists units produced, sold, or installed per period, aka cohorts. The body of the table lists the numbers of failures or “returns” in each period from successive cohorts.
Table 1. Grouped ships and returns counts in a “Nevada” table
Life data | “Ships” | Period 1 | Period 2 | Period 3 | Period 4 | Period 5 | Period 6 | Etc. |
Period 1 | 47 | 1 | 3 | 7 | 8 | 13 | 5 | |
Period 2 | 41 | 4 | 3 | 4 | 7 | 6 | ||
Period 3 | 45 | 2 | 4 | 9 | 6 | |||
Period 4 | 39 | 1 | 6 | 4 | ||||
Period 5 | 43 | 2 | 6 | |||||
Period 6 | 48 | 1 | ||||||
Etc. | Etc. |
The nonparametric maximum likelihood reliability estimator from table 1 data is the Kaplan-Meier estimator. Its properties are well known, including its uncertainty. The grouped failure counts in table 1 are sufficient but not necessary.
What if you don’t have the grouped failure counts in table 1? The bottom row of table 2 contains the failure counts “Returns” (complaints, repairs, failures, replacements, or spares sold) from each period from table 1. Table 2 contains sufficient data to make nonparametric maximum likelihood reliability estimates.
Table 2. Same as table 1 without lifetime failure counts by cohort, but with period returns…
Ships | Period 1 | Period 2 | Period 3 | Period 4 | Period 5 | Period 6 | Etc. | |
Period 1 | 47 | ? | ? | ? | ? | ? | ? | |
Period 2 | 41 | ? | ? | ? | ? | ? | ||
Period 3 | 45 | ? | ? | ? | ? | |||
Period 4 | 39 | ? | ? | ? | ||||
Period 5 | 43 | ? | ? | |||||
Period 6 | 48 | ? | ||||||
Etc. | ||||||||
Sums | 1 | 7 | 12 | 17 | 37 | 28 |
Why estimate reliability from table 2 data? Because you may not have lifetime failure data in table 1; because it costs too much to collect, because lifetime data contain too many errors or missing data, or because you might want the estimator for parts used in several products, or for parts, not products tracked by name and serial number.
Table 2 ships and returns counts are required by Generally Accepted Accounting Principles (GAAP)! You may have to work a little to get the table 2 data from calendar period revenue and cost accounts. Revenue is sales times price, so revenue contains information on product installed base: production, ships, sales, installations, etc. Warranty cost is field services times costs per service, and field services or spares usage provide the bottom line in table 2. After warranty expirations, replacements or spares usage continue to provide period counts in the bottom line.
What’s the catch? There is less information in table 2 than in table 1, and that is evident in the uncertainty in reliability estimates from table 2 compared with the uncertainty in reliability estimates from table 1. Is the cost of tracking products by serial number to get lifetime data worth the cost of the greater uncertainty? Is the cost of a little work to fill table 2 worth the cost of no knowledge of field reliability at all?
Figure 1 shows the nonparametric maximum likelihood Kaplan-Meier (K-M) and from ships and returns (S&R) counts for 18 periods.
The estimators from ships and returns counts have no sample uncertainty, because ships and return counts are population data! Fisher information and Cramer-Rao lower bound give variance of maximum likelihood estimator, and bootstrap gives confidence limits on actuarial forecast (expected demand) and prediction limits on demand random variables for fill rates and stock levels.
Consumer Bills of Rights entitle consumers to product information, which I interpret to mean products’ field reliability, preferably without unwarranted assumptions. Few companies have been willing to share, so I offer to estimate it for them, from their ships and returns counts.
Would you like use your products’ and their parts’ age-specific field reliability and use it to improve design, process, and service: better than traditional methods, best-in-class methods, or nothing at all? Send data to pstlarry@yahoo.com, describe it, and ask questions, and I will send back workbooks and answers as well as I can. Alternatively, use the spreadsheet “NPMLE” that made the estimates in figure 1 from data in tables 1 and 2 in https://sites.google.com/site/fieldreliability/home/list-of-files/.
In future articles, I will describe fun with field reliability: Firestone tires, Apple computers, Tesla battery, drive unit and charger, pacemakers, hard-disk drives, COVID-19…
PS In 1990, Mike Johanns, group leader, regarded Apple parts’ return counts as outputs from an M/G/infinity service system. He told me to do a literature search for “statistics and self-service systems.” The only hit was my article, M/G/Infinity Service Distribution – Field Reliability (google.com).
Leave a Reply