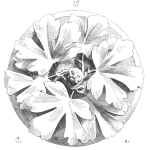
The title was inspired by Rupert Miller’s report “What Price Kaplan Meier?” That report compares nonparametric vs. parametric reliability estimators from censored age-at-failure data. This article compares alternative, nonparametric estimators from different data: grouped, censored age-at-failure data vs. population ships and returns data required by generally accepted accounting principles. This article compares data storage and collection requirements and costs, and bias, precision, and information of nonparametric reliability estimators.
Story Time
Nonparametric reliability estimation doesn’t require as much data as you think. If you have all ages-at-failures, then use the empirical distribution function reliability estimate; each observed failure has equal probability.
“But, we can’t wait for everything to fail!”
Then use the Kaplan-Meier reliability estimator with censored ages-at-failures and survivors’ ages.
“But we only have failure counts grouped by age interval.”
Grouped failure and survivor counts are sufficient for the Kaplan-Meier estimator too. Of course, the estimator is as coarse as the data.
“But we can’t track parts by serial number. It’s too expensive and full of errors. What if there’s no age-at-failure data?”
You don’t need to track every product or part by name or serial number. Ships and returns counts, required by the IRS and generally accepted accounting principles, are statistically sufficient to estimate age-specific reliability, from population data.
“But what’s the penalty for using ships and returns counts?”
Yes, there’s less information in ships and returns counts data than in ages-at-failure data. And you might have to do a little work! Get ships, installed base by ages, from sales and BoMs and get returns counts from service and warranty records; e.g., complaints, failures, repairs, spares sales, etc.
Which would you prefer: grouped, censored lifetimes (table 1) or population ships and returns counts (table 2) required by GAAP? Obviously table 1 contains more reliability information than table 2. But at what cost? Lifetime data requires tracking every product or part by name or serial number. What if table 1 was only a sample and table 2 was population data?
For Example…
Table 1 shows some typical field reliability data, ships (production, sales, installations, etc.) and grouped failure counts in a “Nevada” table. (The body of the table looks like Nevada on its side.) Ages at failures are known if products or parts are tracked by name or serial number. Survivors’ ages are known from ships minus failures. The Kaplan-Meier nonparametric maximum likelihood reliability estimator (KM npmle) is convenient, easy to use, and preserves all information in the data [Miller, George 2005]. (Entropy defines minimal data storage requirements and measures information content.)
Table 1. Ships and grouped failure counts by week of failure
Week | Ships | 9901 | 9902 | 9903 | 9904 | 9905 | 9906 | 9907 | 9908 | 9909 |
9901 | 530 | 0 | 1 | 2 | 2 | 2 | 3 | 2 | 2 | 3 |
9902 | 1290 | 1 | 2 | 4 | 4 | 6 | 4 | 5 | 5 | |
9903 | 3362 | 3 | 7 | 14 | 16 | 12 | 12 | 13 | ||
9904 | 4071 | 3 | 9 | 18 | 13 | 17 | 13 | |||
9905 | 5966 | 7 | 12 | 23 | 26 | 20 | ||||
9906 | 7516 | 9 | 16 | 33 | 24 | |||||
9907 | 8174 | 7 | 16 | 40 | ||||||
9908 | 10235 | 9 | 23 | |||||||
9909 | 12578 | 9 | ||||||||
Sum | 0 | 2 | 7 | 16 | 36 | 64 | 77 | 120 | 150 |
Suppose all you knew were weekly ships and returns, the column sums of failures per calendar accounting interval (bottom row of table 1). Table 2 shows weekly ships and failures, without telling which week failures were shipped. Ships and failure counts are in data required by the IRS and by generally accepted accounting principles, and they are statistically sufficient to make nonparametric estimates of age-specific field reliability.
Table 2. Ships and failure counts by calendar week, without telling which week failed unit were shipped
Week | Ships | 9901 | 9902 | 9903 | 9904 | 9905 | 9906 | 9907 | 9908 | 9909 |
9901 | 530 | |||||||||
9902 | 1290 | |||||||||
9903 | 3362 | |||||||||
9904 | 4071 | |||||||||
9905 | 5966 | |||||||||
9906 | 7516 | |||||||||
9907 | 8174 | |||||||||
9908 | 10235 | |||||||||
9909 | 12578 | |||||||||
Sum | 0 | 2 | 7 | 16 | 36 | 64 | 77 | 120 | 150 |
Data requirements
Tracking auto parts by serial number used to require a mainframe computer; but tracking ships and returns requires a PC. There were ~4000 parts per ~6 million Fords under warranty. With 8 bytes per part number, at least 17 bytes per VIN (typically ~55 bytes), and two bytes for age, that’s 600Gb. (Not all that storage is needed until failure.) Ships and returns counts require a byte for vehicle ships, a byte per part for returns each month, plus bills-of-materials. That’s less than 1Mb.
Accuracy, precision and information
Estimators using ages-at-failures contain more information than estimators using ships and returns counts, but actionable features remain apparent in nonparametric estimators using ships and returns counts. All three max likelihood estimators (empirical from complete failure data, Kaplan-Meier from censored data, and maximum likelihood from ships and returns counts) are asymptotically unbiased. Their precision and information have the same order of magnitude.
Figures 1-3 compare bias, precision, and entropy of estimators: empirical distribution from all ages-at-failures (blue bars), the Kaplan-Meier (red bars) from censored ages-at-failures, and the maximum likelihood estimate from ships and returns counts (yellow bars).
There are four clusters of the three bars in the figures. The four clusters are results of simulated, censored samples from:
- Weibull distribution with decreasing failure rate (DFR)
- Weibull distribution with increasing failure rate (IFR)
- discrete distribution with DoAs, constant failure rate with ~80% survive
- a discrete distribution with DoAs and constant failure rate with~50% survive. The vertical axis is linear for all three figures: bias, precision and entropy.
The Weibull DFR distribution with α = 10 (scale parameter) and β =0.5 (shape parameter) had all but 35% observed failures. The Weibull IFR distribution with α = 50 and β = 1.5 had ~90% survive. The third, a discrete distribution , had ~80% survive. The fourth, a discrete distribution, had ~50% survive.
Figure 1 shows the bias from Weibull samples and from ships and returns counts are about the same.

Figure 1. Bias of Kaplan-Meier (red bars) and ships and returns counts estimators (yellow bars) are close. Complete failure data reduces bias of empirical distribution (blue bars) compared with the other estimators’ biases.

Figure 2. Precision = sums of squared errors. Ships and returns (yellow bars) give a little less precision from a lot less data. The precisions of the estimates from ships and returns counts are worse. No surprise.

Figure 3. Entropy (inverse information) relative to true distribution shows ships and returns counts estimators (yellow bars) can be better (4th cluster). The inverse information in ships and returns is less than life data but the disadvantage decreases as the censoring increases.
Rupert Miller Comparison
Professor Miller compared estimators’ asymptotic efficiency, measured by the reciprocal of the alternative reliability estimators’ variances at specified age t. The asymptotic variance of the Kaplan-Meier reliability estimator R(t) at age t is Greenwood’s formula,
VAR[R(t)] = R(t)2*SUM[d(j)/[d(j)*(r(j)d(j))],
where d(j) is the number of failures at age j, r(j) is the number that survived beyond age j, and the sum is over all failure time less than or equal to age t. The asymptotic variance of other distributions’ maximum likelihood reliability estimators was computed from their Cramer-Rao parameter variance bounds [https://en.wikipedia.org/wiki/Cram%C3%A9r%E2%80%93Rao_bound] by the Delta method [Miller].
The asymptotic variance of the maximum likelihood reliability estimator from ships and returns counts depends on its likelihood function, L = PRODUCT[Poisson(λG(t(j))], where j is the age index, λ is the ships rate and G(t(j)) is the service time distribution of an M(t)/G/∞ self-service queuing system (with Poisson inputs) and service times (Reliability R(t) = 1G(t).) corresponding to the reliability function R(t) being estimated [George, 1973, 1999]. The variance of the maximum likelihood reliability estimator is (1/n)*I-1(R(t))*(f(t))2. I-1(R(t)) is the inverse of the information matrix, E[2lnL/((i)(j))], where the (.) are the reliability estimates at ages failure times I and j.
The asymptotic efficiency of the Kaplan-Meier estimator relative to the maximum likelihood estimator from ships and returns is Greenwood’s formula divided by the variance estimator, (1/n)*I-1(R(t)*(f(t))2 . I use Mathematica, but the figures were prepared using the bootstrap estimator of variance, popularized by Rupert Miller’s student Bradley Efron [https://en.wikipedia.org/wiki/Bootstrapping_(statistics)].
Recommendations
Your company accounts for sales (ships), BoMs tell which parts and how many go into each product (installed base by age), and service, spares sales, and warranty actions account for returns. Accountants get in serious trouble for falsifying data. Ships, returns counts and BoM data is sufficient to estimate age-specific field reliability for every product or service part ever complained about, failed, serviced, replaced, or returned.
If you would like to learn more about the efficiency of nonparametric field reliability estimation from ships and returns counts, please see https://sites.google.com/site/fieldreliability/random-tandem-queues-and-reliability-estimation-without-life-data and read the *.pdf or *docx [George 2019].
If required, track safety-critical components by name and serial number, carefully, and use all failures and survivors’ ages. The FAA requires tracking approximately 75 fracture-critical parts per aircraft. The FDA requires tracking all implantable medical devices by serial number. If not required, don’t start. Use ships and returns counts. That data is free!
References
Rupert G. Miller, Jr., 1933-1986: A Tribute. Statist. Sci. 6(1): pp. 98-99, February, 1991, DOI: 10.1214/ss/1177011944
Miller, Rupert “What Price Kaplan-Meier?” Biometrics, vol. 39, pp. 1077-1081, 1983
George, L. L., “Estimation of a Hidden Service Distribution of an M/G/∞ System,” Naval Research Logistics, 20: 549–555. 1973, DOI: 10.1002/nav.3800200314, https://sites.google.com/site/fieldreliability/home/m-g-infinity-service-distribution
George, L. L. “Field Reliability Without Life Data,” ASA QP and SPES Newsletter, Vol. 5, No. 2, pp.13-14, http://www.amstat-online.org/sections/qp/1299newsletter.pdf, 1999
George, L. L., “Random-Tandem Queues and Reliability Estimation, Without Life Data,” Dec. 2019, https://docs.google.com/viewer?a=v&pid=sites&srcid=ZGVmYXVsdGRvbWFpbnxmaWVsZHJlbGlhYmlsaXR5fGd4OjU1NTQwNTJhNDkxNWJlNGM
Solid post. I like the nonparametric line of thinking.
Thanks, although nonparametric statistics is a way of ->avoiding<- thinking:
Thinking about what distribution fits?
Wondering what physical justification is there for a parametric distribution?
Concerned about modeling error am I inducing by assuming a parametric distribution?
Uncertainty about future if reality changes? [thanks to Karl Rove]