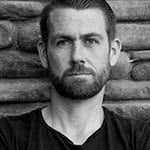
When I was working at a university, I was involved in a conversation with a representative of an energy company. He was having all manners of problems with a valve. It was failing too often. He wanted us to look at what we could do in terms of optimizing the preventive maintenance (PM) or servicing regime to hopefully fix these problems. But … there was a catch.
He had heard about ‘deep learning’ and ‘artificial intelligence’ from another university. And he wanted some of it.
In fact, that was pretty much all he wanted.
We talked about other options, like a Failure Reporting and Corrective Action System (FRACAS) which would allow his organization to examine failures to better understand their failure mechanisms – and by extension inform a better PM regime. We talked about Mean Cumulative Functions (MCFs), modelling Rate of Occurrence of Failure After Servicing (ROFAS) and other tools with proven track records that use existing data.
He wasn’t interested.
And neither were the professors on either side of me. This ‘deep learning’ and ‘artificial intelligence’ stuff sounded like great research opportunities that could bring in more money.
So what is wrong with using ‘deep learning,’ ‘artificial intelligence’ or ‘machine learning?’ Nothing … if they are used at the right time.
Machine (and deep) learning involves computers going through massive amounts of data to identify patterns or other things that might be useful. For example, we can get lots of different people to write down the digits zero through to nine on a piece of paper. We can then show each digit on each piece of paper to a computer using a camera. If we have lots of people showing the computer lots of numbers, machine learning can identify common patterns across digits so that it can automatically recognize (for example) handwritten postal codes on envelopes that allow automated sorting. This is very, very powerful.
Machine learning is seen as a part of artificial intelligence. And in its simplest form, artificial intelligence is all about trying to program a computer to mimic how humans learn.
Sounds great … right? Well, there is a problem with this approach.
There are humans everywhere who are already built to learn.
So let’s go back to the valve I talked about at the very start. Let’s say that the valve was failing due to corrosion. If a human examined a failed valve, they should be able to identify corrosion as the main failure mechanism. And there are lots of textbooks out there with fantastic corrosion models that our human could use to tailor a PM regime … or better yet, work out how to redesign the valve to increase reliability.
But when it comes to artificial intelligence or machine (deep) learning, our computers will need HUGE amounts of failure data to identify a trend that they may or may not be able to recognize as something that could be explained by corrosion.
Computers are different to us. Their overwhelming strength is their ability to sift through HUGE amounts of data that would otherwise overwhelm our human brains to identify trends we can then use to make better decisions. But they need HUGE amounts of data – which is always expensive when it comes to reliability. They need HUGE amounts of data because they haven’t gone to school, university, or spent their entire lives trying to figure things out. Data and experience contain information. No experience means you need more data.
Our (human) overwhelming strength is to be able to deduce or otherwise reason what might have caused something. Like Sherlock Holmes. We started perfecting this the day we were born. And we are so good at it, that deducing what caused something to happen can take seconds. It’s called ‘causality.’
So when do we use artificial intelligence or machine (deep) learning?
When we have exhausted our human efforts.
Before we get wowed by artificial intelligence algorithms and machine (deep) learning software, we need to use our ‘human computers’ first. They can identify that wonderful thing called ‘causality’ because they have spent their entire life becoming good at it.
Artificial intelligence and machine (deep) learning are fantastic tools. But you need to focus on the problem you are trying to solve and not fall in love with a tool. A six degree of freedom milling machine is a very sophisticated tool. But sometimes, you just need a screwdriver.
What about you? Have you had any experience where an amazing new tool that has been forced upon you or your team where trusting a human would have been a better option?
Leave a Reply