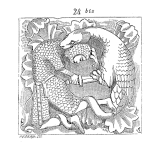
ASQ Reliability Division published “Credible Reliability Prediction” (CRP) in 2003. Harold Williams, Reliability Division monograph series editor, wrote, “[CRP] …delineates statistical methods that effectively extend MTBF prediction to complex, redundant, dependent, standby, and life-limited systems… This is the first text that describes a credible method of making age-specific reliability predictions…. This monograph presents insights and information inspired by real applications and [still] not covered in contemporary reliability textbooks.”
In 2004, Fred Schenkelberg invited me to present CRP to Hewlett-Packard; so I did. Thanks Fred. Did anyone at HP actually do CRP? I did, for Brocade, Agilent, Violin Memory, and others.
We need age-specific reliability predictions, not just MTBF predictions. We want reliability predictions for systems made complex by redundancy, new designs, repair, dependence, and maintenance. We want these predictions for:
- Evaluating alternative designs and allocating reliability
- Estimating warranty risk and setting warranty reserves
- Providing early warning and statistical process control of excessive infant mortality or premature wearout
- Forecasting service and spares requirements
- Verifying improvements and evaluating and verifying fixes
We are paid for accurate, precise, and defendable predictions that give just cause for design, process, and service actions. So make the best reliability predictions possible, objectively, accurately, and precisely, using all available, relevant data and information (Walter Shewhart’s rule #1).
The Field Determines Reliability!
“Credible Reliability Prediction” is not MTBF prediction a la MIL-HDBK-217, Bellcore TR-332, other vendors, and a freebie [George]. CRP predicts a credible nonparametric reliability function for a new product. What makes it credible?
CRP uses field failure rate (actuarial) estimates a(t;OLD) of older, comparable parts and products, their MTBF predictions, and an MTBF prediction of the new product to predict reliability R(t;NEW) = exp(-INTEGRAL[a(s;NEW), s=0 to t]), where a(s;NEW) is the new product failure rate function. Generations of products have similar failure rate functions, because factors such as process, shipping, installation, training, environments, and customers remain the same even though designs may change. New products may even use old parts for which you may already have estimates of their field reliability and failure rate functions. For example, a credible a(t;NEW) prediction is a(t;OLD)*MTBF(OLD)/MTBF(NEW)), for one new and one old, comparable product.
Adjustment of failure rate functions is not an original idea. Real statisticians call this a “proportional hazards” model, Proportional hazards model – Wikipedia.
Resistance to change?
MTBF-prediction armchair exercises require only looking up part FITs (Failures In Time, also Failures In Thousands of millions of hours), multiplying by part counts and (fudge) p-factors, adding the multiplication products, and inverting the sum. This simplicity has such strong appeal that the parts-count method persists despite out-of-date FITs data, invalid model assumptions, irrelevance of MTBF during useful life, and lack of credibility [Pandian et al., EPSMA, Jiang and Chen, George and Langfeldt]. MTBF prediction, given a credible reliability prediction R(t;NEW), is easy: MTBF = INTEGRAL[R(t;NEW), t=0 to infinity, life limit, or useful life].
Credible Reliability Prediction methods apply to complex, redundant, dependent, standby, and life-limited systems, using observed reliability of comparable products or parts. Finally, CRP adapts insurance credibility theory to update predictions as new-product user data becomes available.
Try Credible Reliability Prediction?
URL Credible Reliability Prediction – Field Reliability (google.com) is the preface to CRP. Try the do-it-yourself spreadsheet CRPExamp.xlsx implementation in the List of Files. Take a look at the 2nd edition of Credible Reliability Prediction, CREDRP2020.PDF, at the bottom of that web page. I will help use it. Next article will be about the “User Manual for CRP.”
Fred Schenkelberg suggests that you need to let me know what you think of these reliability-statistics articles and their applications. Comments get emailed to me, and I appreciate them.
References
Guru Prasad Pandian, Diganta Das, Chuan Li , Enrico Zio, and Michael Pecht, “A critique of reliability prediction techniques for avionics applications, Chinese Journal of Aeronautics, Vol. 31, issue 1, Jan. 2018, pp. 10-20
EPSMA, “Guidelines to Understanding Reliability Prediction,” European Power Supply Manufacturers Association, June 2005
Mingxiao Jiang, Weiqiu Chen “Integrated Approach for Field Reliability Prediction Based on Accelerated Life Testing,” Quality Engineering, 27:317–328, 2015
George, L. L., “MTBF Prediction Workbook,” ASQ Reliability Review, Vol. 24, No. 1, pp. 18-23, March 2004
George, L. L. and Eva Langfeldt, “MTBF vs. Age-Specific Reliability Prediction,” ASQ Reliability Review, Vol. 21, No. 2, June 2001, pp 13-15. Translated and published in Gestion de Activos Industriales sometime after Sept., 2001
I like how you stated the differences between credible reliability prediction and MTBF prediction. It was very helpful reading about the CPR and how it measures reliability. I have been wanting to learn about the CPR as I usually use MTBF prediction.
Sorry this reply is so long in coming. I am happy that you recognize the difference between MTBF and age-specific reliability predictions.
There is a lot of criticism of MTBF predictions, and I am working on a revised version of MIL-HDBK-217G (George) that uses Paretos to improve MTBF predictions.
I also offer help to make nonparametric estimates of field reliability and failure rate functions to help make Credible Reliability Predictions, without lifetime data [https://drive.google.com/file/d/1vxzrQUQKciZ1uyB1ZF_O-m4VcK6oVZe8/view/]
[https://drive.google.com/file/d/1za5KT_qsF2sCSzGO7xi2EoHONBz2PwtZ/view/]