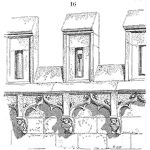
I have been working with clients recently who are keen users of FMEA. Getting engineers to contribute potential failures and their causes is not a problem with these clients, but ensuring that actions are correctly identified and followed up is not so easy. So, what goes wrong? What differentiates a good FMEA from a great FMEA?
Define the Product or Process
Effective FMEA depends on a clear understanding of the product or process under analysis.
What are its Functions?
It never surprises me now how product functions are incompletely defined at the start of an FMEA. How much, within what limits, when, in what sequence, etc. are all required to fully define a function. And, of course, we need ALL functions. We often forget installation requirements, maintenance / service, how to respond to problems, etc. So, before we delve deeper into what can go wrong with FMEA corrective actions, get the functions sorted!
Failure Modes & Causes
My experience has been that filling out the failure modes and causes is the easiest part of the process, provided that the facilitator ensures that all aspects of a function are addressed. A function can be “not achieved”, “partially achieved”, “achieved too early / too late”, “achieved differently”, etc. So, get these and engineers seem to have an inbuilt ability to offer up potential causes. Just be thorough!
Failure Effects
Failure effects are not usually an issue for good FMEA development either, provided that all potential effects are listed. Consider where the product or process will be stored, shipped, installed, used, serviced; by whom, with whom nearby? The FMEA will have a defined scope, and this will limit how far failure effects will be developed.
Scoring
Scoring is where I first see things going wrong. Severity is usually OK; just go with the worst possible outcome. Detection also, providing that the scenario and scope for the FMEA are clear.
But, scoring of the likelihood of occurrence has several problems.
Data Uncertainty
The first problem that I have seen is in applying optimistic scoring for the likelihood / probability of occurrence. In many cases, particularly at the early stages of a project, the likelihood of failure is not backed by clear evidence. We may “think” or “hope” that occurrence of a particular failure is “unlikely” but just how sure are we?
- A previous generation product may have good data that a particular critical failure is rare. But the new product has a slightly different configuration. I’ve seen this scenario where the project team “assumed” that the previous good results would carry over. The FMEA entry did not make it onto the critical items list and no action was taken to obtain data that the configuration change did not result in a higher risk of the critical failure. …The outcome was a high field failure rate of a critical failure.
- A new design may be without data to support a likelihood of occurrence score. What should be done? Use a data handbook or other industry data? Sources such as these give a number, but how much trust should we place in this?
These 2 scenarios, where “evidence” is left unvalidated, give a false indication of confidence.
In response, I have seen 2 approaches:
- Artificially increase the occurrence score. This will often result in the potential failure being on the critical items list and being in the top risks. However, doing so can also flood the critical item list and dilute attention from other risks.
- Separately highlight data uncertainty, and take “corrective” action to validate or at least bound the uncertainty.
I suggest that both approaches have their merits. If you don’t have a close handle on the probability of failure, then the result could be worse than you think. Hence, artificially increasing the score is perhaps reasonable. On the other hand, doing so does not necessarily address the real issue: inadequate data. But to simply validate the data does not direct action to a potentially high-risk failure.
I recommend a combination of the 2 approaches.
Input a pessimistic occurrence score, based on what data you have. Do not assume the best outcome, but rather acknowledge evidence pointing to a less advantageous outcome. This isn’t an “artificial” score increase but rather one based on an honest assessment of the data available. Maybe early failures from the previous design were more frequent than later in its production, after modification and manufacturing improvements.
Maybe this acknowledgement prompts corrective action to improve the new design to specifically avoid the failure causes found during early production of the previous design.
But, in addition, lack of data validation should also be addressed. What is required is to be able to set limits on the assessment. How bad could the rate of failure be and be acceptable? At what point in the development does this need to be settled? As a general rule, design improvements should be applied first before validation: why test what you know is going to fail? But maybe the design corrective action would be costly and time-consuming, and maybe it isn’t required. In which case, validation of existing assessments would come first.
It is only by considering both design improvement and data validation as potential corrective actions that the correct project choice can be made. FMEA is best when it considers both.
Cost of Failure
We have already noted that FMEA should, depending on the Scenario defined for the analysis, consider the full lifecycle of a product and the full range of those systems and people interfacing with a product or process. However, when it comes to scoring likelihood of occurrence, I sometimes see confusion. Of course, every good Standard describing FMEA calls for a scoring rubric to be defined appropriate to a particular project. However, because we measure reliability in different ways, our data may not all be in the same format. Failure rate; life; Weibull beta; maybe some manufacturing quality metric? And the data may describe installation risks (a “one off”), early life failure, wear-out in later life, or response to specific events.
The scoring rubric, although project specific, may still be generic because it needs to cover all these scenarios. As we know, everything will fail eventually, given enough time and operation. So, for a product with a 5-year life, does one score a failure rate of “x per year” in terms of 5x (5 years) or look to the risk of failure in any given year? An early-failure risk (installation and, say, the first year) could be high impact and be more critical to the project than a similar FMEA score after 5+ years.
My experience is that scoring rubrics can fail to offer enough guidance, and the project manager or facilitator does not clarify where and when risks are most critical to project success. A similar size risk (numerical score) based only on simplistic scoring rules could have different impact.
My recommendation is to review the scoring rubric before each FMEA. Discuss different scenarios, and clarify the rubric to ensure that a good balance is achieved. Consistent scoring, yes! Scoring to reflect the real impact on the user / customer / business, yes also! Scoring based on a full appreciation of the Cost of Failure!
Implement Great FMEA
I wish you all well in your effective use of FMEA.
FMEA is a great tool. Let’s not use it to give ourselves a false sense of security or as a vehicle to declare that the sky is falling in. FMEA needs to focus the right action where it is most needed.
Please contact me via my Contacts page or my website www.lwcreliability.com.
Leave a Reply