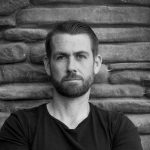
I am constantly confronted by students, reliability engineers and other people banging fists on tables and saying …
… 89 percent of failures are random …
Firstly, 100 percent of failures are random. It’s just that there are lots of textbooks and experts telling us that a ‘random’ failure is one that happens irrespective of age. That is, a failure with a constant ‘failure rate’ where the item in question doesn’t appear to age or wear out.
This is complete rubbish. For example, if something fails due to fatigue cracking (which is a classic ‘wear out’ failure mechanism that becomes more likely when things age), we can’t say with absolute precision when it will fail. We might be able to model the failure mechanism and come up with a really good guess, but there will still be some variation in when seemingly identical components fail due to things like fatigue. This, by definition, makes wear out failure ‘random.’
The quote above comes from an often-cited 1978 study completed by F. Stanley Nowlan and Howard F. Heap (Nowlan and Heap). They both worked at United Airlines, so their focus was obviously on aircraft in the United Airlines fleet. The figure of ‘89 percent’ comes from their report and has been trumpeted as some laminated ‘golden’ figure across many industries for many years.
But it’s wrong. Here’s why.
Let’s start with Nowlan and Heap’s analysis
It is more than a little concerning.
For example, their report included a chart that showed failure data for 50 Pratt & Whitney JT8D-7 engines over the first 2 000 hours of use. Of the 21 that failed before 2 000 hours, Nowlan and Heap concluded that because there was no ‘clustering’ around the average failure age of those 21 engines (around 861 hours), there is no discernible trend regarding failure rates. Or in other words, the engines weren’t accumulating damage.
Really? A quick Weibull analysis suggests that of those 21 failed engines, the shape parameter was around 0.8 to 0.9 with a relatively wide margin of error. For those of you who aren’t statistical gurus, any value less than one (1.0) suggests that something is wearing in. But 0.8 to 0.9 is not that far off 1.0, so let’s extend a substantial benefit of doubt and say that a constant failure rate is a possible characteristic of those 21 engines.
So what about the other 29 engines that were still working at 2 000 hours? If these engines are accumulating damage (or wearing out), you would expect to see that most prominently in the oldest engines. In other words, the last engines to fail would be the ones to demonstrate if something is wearing out (or not). But because the data set has no failure data for the last 58 percent of engines (that were still working at 2 000 hours), how can anyone claim that they ‘know’ those engines aren’t accumulating damage and would have clear signs of wear out or increasing failure rates if we were to keep testing them beyond 2 000 hours?
You can’t. And it beggars belief given we know the myriad of failure mechanisms that involve the accumulation of damage in aircraft engines like fatigue, creep, and corrosion.
But it gets worse.
Sorry … but we need to touch on statistics a little bit more.
Nowlan and Heap use a different set of data for the Pratt & Whitney JT8D-7 engines to create a reliability curve. A reliability curve is the percentage of items you expect to still be working after a period of time. It usually starts at 100 percent and decreases over time as the probability of failure increases.
But Nowlan and Heap do something a little different. They are not focused on the age of the engine, in their opening discussion on aircraft component reliability. They are focused on the time each engine has spent since the last shop visit. And each engine visits the shop every 1 000 hours.
So the data Nowlan and Heap uses is not based on actual engine age, and there is no data that extends beyond 1 000 hours. But this does not stop them from creating a reliability curve that goes beyond 1 000 hours.
And … there is a HUGE problem with the curve they came up with. The curve is very straight (see the red line in the illustration below) as it goes from 100 percent down to 0 percent over a range of 4 000 hours. And (here is the really crazy bit), if you actually analyze this reliability curve, it implies a failure rate that has to increase to infinity at 4 000 hours ‘to work’ (see dashed red line in the illustration below).
Even the most junior reliability engineer will tell you this type of reliability curve does not exist – especially if you are arguing that the engine in question has a constant failure rate. The only thing we know from the report is that 69.2 percent of the engines had not failed by 1 000 hours after the last shop visit. So if we assume a constant failure rate (as Nowlan and Heap claim they have done), then the correct reliability curve is one based on what we call the ‘exponential distribution’ (blue line in illustration above.) And you can see how different that curve is. It implies (amongst other things) that 22 percent of the engines would still be working at 4 000 hours, where Nowlan and Heap claim a figure of zero percent.
A more detailed explanation of the statistics is beyond this article … but suffice to say that those differences are kind of a big deal and make it difficult to believe that the conclusions made in this report are credible.
So nothing adds up. But it doesn’t stop Nowlan and Heap from using the clearly wrong reliability curve to come up with meaningless statistics that supports their claims about aircraft component reliability.
If I had to have a guess at the model Nowlan and Heap use … it would simply be ‘the straightest line possible.’ Which is something you can’t do in reliability engineering, as we know how failure models influence things like reliability curves. Like the exponential distribution.
And of course, with no data going beyond 1 000 hours, we can’t say with any certainty that those engines would not be wearing out at any point beyond that cutoff time.
So what about this 89 %?
Nowlan and Heap came up with six (6) categories of failure rates for aircraft components, and assigned a percentage breakdown for each (as illustrated below).
The small charts on the right represent the failure rate characteristics for each category, with the blue lines representing failure rates that increase over time (indicating the accumulation of damage or wear out). The red lines represent failure rates that do not (indefinitely) increase over time.
But the problem for me is … given what I saw in their data analysis of their Pratt & Whitney JT8D-7 engines, I personally can’t put a lot of stock in the way they have come up with the categories above.
At best, these percentages are interesting conversation starters (note that it suggests that 72 percent of components experience wear-in), but I wouldn’t put any stock in these figures being anywhere close to the actual numbers, particularly given that Nowlan and Heap don’t provide much of the raw data their analysis is based on.
Further, given their data is based on lots of parts that are arbitrarily removed and replaced after fixed intervals of usage (this is stated many times), it would be impossible to conclude that this ‘hypothetical 89 percent’ don’t include components that would be wearing out if they were being used for longer periods of time.
But even if their report was ‘right’ … it still wouldn’t be right for you
In 1978, there were around 5 accidents per million flights that involved fatalities. Today, that figure is less than 0.5. So there is around a factor of ten improvement in aircraft reliability. So the data used by Nowlan and Heap would not be relevant to any aircraft today.
But that data is certainly completely irrelevant to any other industry, regardless of timeframe. The aircraft industry is heavily regulated, with lots of structural aircraft components like spars being routinely inspected and maintained. Now structural elements like spars will eventually degrade, but they are designed to not degrade significantly throughout the life of an aircraft. This means, we would not expect to see them wear out, even though they eventually would, decades or centuries from now (long after the aircraft has been withdrawn from service). This is much like the chassis of a car, which is made from steel and eventually will corrode away. It’s just that the chassis is so strong and corrosion is well understood, that we know the chassis will outlast the typical lifespan of most vehicles. So they too will not ‘appear’ to degrade during the life of a vehicle.
There are lots of components in aircraft that are designed to ‘outlast’ the plane, which are routinely inspected anyway, which give (at least some) impetus to the idea that lots of things have constant failure rates. And of course, Nowlan and Heap routinely describe about their data sets are at best ‘short in duration.’
So none of the stuff they say (even if it was completely correct) applies to your electronic component manufacturing facility, nuclear generation plant or whatever it is you are responsible for … BECAUSE THEY ARE NOT UNITED AIRLINES AIRCRAFT CIRCA 1978!
So the takeaway is?
You have to study YOUR machine, system, plant or whatever it is whose reliability you are responsible for. One thing that Nowlan and Heap are right about though is you shouldn’t simply service or conduct preventive maintenance without thinking about it. Unnecessary maintenance always incorporates what we call ‘maintenance induced failures’ which involves a temporary spike in failure rates. This spike will be higher if the quality of your maintenance is poor, but there will always be a spike. So you don’t want to do maintenance unnecessarily.So before you start spruiking ‘89 percent of failures are random’ … actually focus on how your system, product or item fails. And this can give you a huge advantage over competitors
Excellent. Well placed, the concept. We can’t use N&H curve any more in most of the cases, including component level Live Data, while for human case,bits always good to have similarity in understanding concept, but for machines, failures do not follow bath tub curve, varies machine to machine, component to component
Thanks for your comment Tarapada. It’s crazy to think that everyone’s machines as of today behave in exactly the same way as commercial airliners circa 1978!
Great article Chris, and one that answers a question that quite often comes up when conducting RCM-style maintenance strategies.
I’ve always explained it to people that the state of airline maintenance around the time of the study was to preventatively replace components at fixed intervals which often left life on the component as it was discarded. This would explain both the high amount of non-time dependent failures (as they weren’t able to accrue hours) and the high infant mortality failures recorded (maintenance errors). I hadn’t realised how they measured component life though, which is definitely a cause for concern as you have stated. Thanks for taking the deep dive into their analysis and showing us the light!!!
Thanks Nik … spread the word! I could be a little blunter and say that people who ‘swear’ by the 89 % rule either (1) aren’t capable of analyzing their own maintenance data, or (2) can’t be bothered. I can’t think of a third option … and I would put a lot of money on most falling into category (1)!