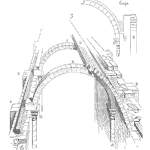
The easy answer is very often. Each time you want to know how long a product will operate. The accompanying question on how well the estimate will match actual performance makes the real answer more difficult.
We regularly and intuitively do reliability predictions all the time. When starting a car at the beginning of a trip, we estimate the ability of the vehicle to complete the journey. When we purchase a phone, we expect it to operate for at least two years (your expectations may differ).
During the design process, we may have formal or informal useful life expectations. It is not knowing if our decisions related to the design will fulfill the lifetime expectations that leads to the desire to know how well the resulting system will operate. We also may need to estimate warranty or maintenance costs, thus knowing what is likely to fail becomes important.
In general, knowing how long something will operate without failure provides the feedback we need to create a viable system that meets our business and customer reliability expectations.
In short, we do reliability predictions regularly to gauge is we are making good decisions.
Reliability prediction methods
Estimating reliability performance may involve a simple engineering guess, little more than a passing assumption to a full-scale study fully characterizing each potential failure mechanism. Every decisions related tot the design of a system involves some level of consideration of future reliability performance.
Every decisions related tot the design of a system involves some level of consideration of future reliability performance.
Engineering judgement
Engineers tend to design away from failure.
The premise underlying this approach is the awareness of potential failure mechanisms. Experience, discussions, and basic risk assessment activities increase awareness. Education may include exposure to material strengths, assembly best practices, and stress & strength information, which provide a foundation for engineering judgment.
At some point, the decision maker realizes the need for better information related to reliability. They may be unfamiliar with the material, desire to verify the new design is better than the previous one, or the perceived consequences of premature failure are too high.
Something triggers the need to predict the reliability performance using a method other than an assumption or engineering judgment.
Material or device ratings
One way a system can fail is the applied stress is larger than the strength of the material or structure.
If the designer initially selects a linear low-density polypropylene with 50°C operating temperature and later learns the intended application will enjoy sustained 60°C temperatures, he should assess the ability of the material to function. In this case, selecting another polymer or possibly a metal would improve the inherent material strength thus capable of operating at the expected temperatures.
The same reasoning applies to electronic components. If the application places 5 volts across a capacitor, select a capacitor with a voltage rating of at least 10V.
In each case, the underlying assumption is the rated ability of the material or component to withstand the applied stress, with an adequate margin, improves the reliability performance. Adding margin is often an initial step when considering the uncertainty of reliability performance.
The process may reduce or minimize early failures, yet doesn’t provide a means to estimate the time to failure distribution.
Parts count predictions
Based on historical databases of reported component failures, or vendor or test data, this prediction method relies on listing the failure rate for each component then tallying for the system failure rate. A common simplification is the assumption that each component is independent and has a constant hazard rate (failure rate does not depend on the age of the component).
Telecordia and other organizations collect component failure information and provide databases of failure rates. Some have adjustments to the failure rate dependent on stress (temperature, voltage, etc.). Vendor supplied failure rate data may or may not have supporting modeling, testing, or field history. It’s worth checking and understanding the assumptions and sources of the data used.
Parts count predictions improve is the design uses fewer components or operates at a lower temperature. Thus the consideration of a parts count prediction, while not very accurate may help the design team improve the system reliability. Another advantage is the ability to create an initial estimate with little more than a bill of material and system architecture.
Dominant failure mechanisms
Some products have one component or failure mechanism that dominates the time to failure distribution. For example, a memory tape drive read/write head has a glass surface that sets the distance between the tape and the magnet. If the distance is not within a very tight set of specifications, the device fails to read/write properly and the system fails.
Each foot of tape pulled over the read/write head removes a small amount of glass and eventually will erode the glass below the minimum specification causing a failure. With normal use, the device will wear out the read/write head well before any other component has an opportunity to fail.
Another example is the brake pad within a car braking system. The pad is designed to abrade with each braking action and it will wear out and require replacement well before any other component within the system. When estimating the time to repair for these systems you may need to only consider the read/write head or brake pad time to failure information.
Field data of similar products
Many products are similar to previous products. If you have access to the field failure information for the similar products, you can use that information to estimate the new system’s reliability performance.
Field data is from actual customers using similar technology in a similar environment and use profile which is often very difficult to replicate with product testing.
A time to failure analysis (Weibull or suitable distribution) provides a means to estimate the time to failure probabilities over a range of durations. If the data provides adequate failure information, you may be able to break down the data to specific subsystems or components, thus providing information on previous design weaknesses.
One technique that may apply when say 80% of a new design is similar to previous products, is to use a simple system model to separate the similar and new elements of a product, then like a parts count method, use field data for those elements that are the same, and another method of prediction for the new elements.
Accelerated life testing
One way to determine the time to failure distribution is via testing. Accelerated testing either uses the device more often or at higher stress than in normal application, thus causing failure to occur much quicker than in normal use.
These tests are often focused on one failure mechanism and can be expensive to conduct.
When possible operating the device more often than operated normally (say a home coffee brewing system which makes an average of 4 cups per day) permits the application of the full range of stresses experienced.
For some failure mechanisms, or devices operated nearly full time, the simple time compression may not be an option. In these cases focusing on specific failure mechanisms may permit the modeling and analysis of elements of a system thus reducing the cost and duration of the accelerated testing.
When a material or element of a product is new and the team has limited knowledge about the time to failure, along with sufficient engineering judgment that the potential failures will occur within or shortly after the expected useful operating life with sufficient uncertainty, then consider conducting an accelerated life test.
Physics of failure analysis
For specific failure mechanisms that have been sufficiently characterized and modeled, you may find you can estimate the time to failure distribution quite well based on the previous work and testing. This approach depends on having the appropriate models that describe the failure mechanism within the use conditions.
Many electronic components and materials have physics of failure models permitting the evaluation of entire circuit boards. DFR Solutions and CALCE have software applications based on current physics of failure models that may be of use.
Summary
Given that we conduct reliability predictions quite often and the need for accurate predictions increases as the importance and complexity of a system increases, the reliability professional should master the range of prediction methods. Furthermore, we should be able to identify the appropriate method for each element of a system.
Having the reliability performance feedback early and often during the system design process provides the team information to base decisions. Not all predictions require the complexity of an accelerated life test, yet a few will.
Balancing the risk of uncertain predictions with the associated importance of the decisions is one way to select the appropriate method and provide adequate reliability predictions.
Related:
Reliability Predictions (article)
ALT and HALT (article)
How to Create an ALT Plan (article)
Leave a Reply