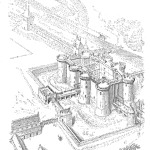
What are Variables Control Charts?
From NIST Engineering Statistics Handbook, section 6.3.2, with edits and additions.
During the 1920’s, Dr. Walter A. Shewhart proposed a general model for control charts as follows:
Let w be a sample statistic that measures some continuously varying quality characteristic of interest (e.g., thickness), and suppose that the mean of w is μw, with a standard deviation of σw. The sample means have an approximately normal distribution based on the central limit theorem.
Taking sample measurements provides a way to estimate the current production output, in this case, thickness. If the mean and standard deviation values remain relatively constant, we can determine the process is in control.
If the sample shows a convincing change versus the expected, we say the process is out-of-control. When a stable process become “unstable” or out-of-control” it is a signal that something has changed and requires investigation and possibly intervention.
Variation
Every process has variation. When the sample measurements indicate a large change, it most likely means the underlying and unknown population has changed. We can expect about a +/-1.5 sigma change in the sample mean with an unchanging or in control process. By convention, when the sample indicates a +/- 3 sigma change, there is only about a 2.5% chance the sample is from the previously stable process.
Thus we place the control limits at +/- 3 sigma from the average of the samples, ˉX. Then the center line, the UCL and the LCL are
UCL=μw+σw
Center Line=μw
LCL=μw−σw
where k is the distance of the control limits from the center line, expressed in terms of standard deviation units. When k is set to 3, we speak of 3-sigma control charts.
Historically, k = 3 has become an accepted standard in industry.
The centerline is the process mean, which in general is unknown. We replace it with a target or the average of all the data. The quantity that we plot is the sample average, ˉX. The chart is called the ˉX chart.
We also have to deal with the fact that σ is, in general, unknown. Here we replace σw with a given standard value, or we estimate it by a function of the average standard deviation. This is obtained by averaging the individual standard deviations that we calculated from each of m preliminary (or present) samples, each of size n. There a couple of ways to estimate standard deviation for control charts and we’ll discuss that in another post.
Looking at standard deviation
It is equally important to examine the standard deviations in ascertaining whether the process is in control. Learn to focus on controlling the standard deviation first, then work on the average. When the standard deviation is out of control the indications about the average may only be a reflection of a change in the standard deviation.
Think target practice
One analogy is in target shooting. First control your process for how you aim thus creating a tight shot group (small standard deviation and stable), then adjust the aim to focus on the target.
That is the basic idea for variables data control charts.
Related:
Selecting Control Charts (article)
8 Steps to creating an X-bar and s control chart (article)
Central Limit Theorem (article)
Leave a Reply