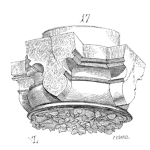
“Component D” had some failures in its first 12 months. How many more would fail in 36-month warranty? ASQ’s Quality Progress Statistics Roundtable published the data and Weibull analysis. The data included left-censored failure counts collected at one calendar time. The Weibull analysis included actuarial failure forecasts. This article describes nonparametric alternatives to Weibull and quantifies extrapolation uncertainty. The nonparametric forecasts are larger than the Weibull forecasts. Alternative extrapolations of nonparametric failure rates from data subsets quantify uncertainty.
Background
Theoretical or physical properties may justify the choice of Weibull or other reliability functions [Brown and Wohletz, Çinlar, Newman et al., extreme-value theory, and http://www.fieldreliability.com/VirtStat.htm]. “But in practice where is the oracle that informs the statistician of the correct choice?” [Miller] Nonparametric statistics make no unwarranted assumptions and preserve all information in data.
Actuarial forecasts require extrapolation of failure rates for installed base older than the age of the oldest failure, regardless of distribution assumptions. Estimating Weibull parameters from 12 months of data and 99.85% reliability, and then using the Weibull failure rate for 36-month forecasts, is extrapolation, strains credibility, and underestimates failures. Extrapolating nonparametric failure rates, based on other, older products’ failure rates, is more credible.
Consumer Bills of Rights entitle consumers to product information that should include reliability and quantification of the uncertainty in reliability estimates, without unwarranted assumptions.
Left-Censored Failure Data
Left-censored failure data results from several causes:
- Prior failure records were unknown due to change of ownership (leased computers, Belgian C130H aircraft) [George 2001 and 2008]
- Failed items were saved and returned in batches (rubber gloves)
- Failures from each month’s installation were collected one day without any record except they occurred prior to the collection day (Component D, table 1)
Component D. Failures could have occurred any time after installation; i.e., their ages at failures are less than or equal to “months in service.” The naive reliability estimator, 1-F(t) = 1-Failed/Installed, is not monotonically decreasing.
Table 1. Component D data and naive reliability estimates
Months in Service | Installed | Failed | 1-F(t) |
1 | 25439 | 1 | 0.99996 |
2 | 25389 | 3 | 0.99988 |
3 | 25183 | 6 | 0.99976 |
4 | 24874 | 10 | 0.99960 |
5 | 24604 | 7 | 0.99972 |
6 | 24355 | 14 | 0.99943 |
7 | 24057 | 11 | 0.99954 |
8 | 23768 | 21 | 0.99912 |
9 | 23389 | 18 | 0.99923 |
10 | 22844 | 30 | 0.99869 |
11 | 22120 | 37 | 0.99833 |
12 | 21069 | 29 | 0.99862 |
Nonparametric Methods
Nonparametric Estimation from Component D Left-Censored Data
The likelihood function and sum of squared errors are
L = PF(t)r(t)(1-F(t))n(t)-r(t) and SSE = S(r(t)-n(t)F(t))2
where n(t) and r(t) are monthly installed base and reported failures of ages t = 1-12 months, and F(t) is the cumulative distribution function. To make monotonic estimates of F(t), substitute F(t) = Sp(s), where p(s) is the discrete probability density function and Sp(s) is summed to age t. Excel’s Solver finds nonnegative p(s) values that maximize lnL and minimize SSE. These nonparametric maximum likelihood and least squares estimates (npmle and nplse) have more likelihood and less SSE than Weibull estimates (figure 1).

Broom charts help show whether failure rate improved, deteriorated or remained the same during production [George]. Although failure rate increases with age, the monthly failure rate broom chart shows nearly the same monthly failure rates for all 12 months of production (figure 2).

Why Weibull?
Here are four reasons for preferring the nonparametric alternative to an unjustified Weibull reliability assumption.
- Alternation is apparent in the naive and nonparametric estimates: especially in figures 2 and 3. This alternation could be due to failure reporting. The Weibull assumption obscures this alternation.
- Rupert Miller says, in comparison with Weibull, “…the asymptotic efficiencies [ratio of variances] of the Kaplan-Meier estimator [nonparametric, right-censored] are low, especially for high censoring proportions or for surviving fractions that are close to one or zero. Low efficiencies for high surviving fractions…are not a cause for worry because the variances of both the Kaplan-Meier estimator and the maximum likelihood [Weibull] estimator are small and the surviving fraction is accurately estimated.” The same is probably true with left-censored data. You might want to read a rebuttal, [Meier et al.].
- Entropy measures the uncertainty of a random variable; less uncertainty is better. Entropy is -Sp(t)ln(p(t), where the sum runs from 1 to 12 months. The npmle entropy is 0.0127, and the mle Weibull entropy is 0.0135. The difference 0.0008 is entropy increase attributable to the Weibull assumption, 6.55%.
- The Akaike Information Criteria (AIC) is a model selection measure that balances overfitting vs. goodness of fit. AIC = 2k-2lnL, where k is the number of estimated parameters, http://en.wikipedia.org/Akaike_information_criterion. The nonparametric AIC is so close to the Weibull, 16.685 vs. 16.683, that nonparametric estimation should be preferred when there is no justification for the Weibull assumption.
Actuarial Forecasts
Actuarial forecasts have been used for more than 50 years for managing expensive gas turbine engines (RAND and the former US Air Force Logistics Command). They suit nonparametric estimates, because they use discrete nonparametric failure rates.
The actuarial forecast is E[Failures] = Sn(t)a(t), where n(t) is the surviving installed base of age t, a(t) is the (discrete or actuarial) failure rate function, and the sum runs over all ages of the installed base in the forecast interval. Table 2 shows alternative forecasts of future failures, from the first 12 months of production, in the remainder of the 36-month warranty. Component D installations stopped after 12 months. Forecasts require extrapolation of failure rates from age 13 to 36 months.
“In extrapolating, use simple functional forms” [Armstrong]. The linear extrapolations (figure 3) of nonparametric failure rate estimates use all 12 failure rates. The Weibull least squares failure rate function estimate is somewhat larger than the mle Weibull [Meeker et al.]. The linear extrapolations of the nonparametric failure rate function estimates yield larger forecasts than the Weibull failure rate extrapolations.

Table 2. Warranty failure forecasts, E[Failures in 36 months|Survival to table 1 ages]
Extrapolation Method | E[Failures|Survival to current age] |
npmle linear extrapolation | 2687 |
nplse linear extrapolation | 2704 |
mle Weibull | 2066 |
lse Weibull | 2495 |
Meeker et al. | 2032 |
Sample Uncertainty
Table 3 shows alternative estimates of the fraction failing in 36 months,
P[Age at failure £ 36 months] = F(36). The npmle extrapolation estimates of fraction failing are larger than the Weibull estimates, even comparing ~90% npmle with the 95% Weibull upper confidence limit (UCL).
RSPLIDA software gives a 95% UCL on fraction failing of 0.014 [Meeker et al.]. This may have been computed using the sample uncertainty estimated from a right- but not left-censored sample, so it probably underestimates. The reason for this claim is that the Fisher information matrix from a right-and-left-censored sample for a Weibull reliability function appears complicated to derive, let alone program, but the right-censored matrix is easy to program [Miller]. The inverse of the Fisher information matrix gives the asymptotic variance-covariance of maximum likelihood parameter estimates. The Fisher information matrix for the left-censored npmle is programmable.
Extrapolating failure rates from data subsets yields 10 estimates of F(36); their maximum is 0.027. This ~90% UCL is computed from all data, all but last month, and so on, as in broom charts, to obtain 10 extrapolations. Extrapolating the failure rates from jackknife estimates yields 11 extrapolations of F(36); their maximum is 0.034. This ~89.1% UCL is computed by deleting one month’s observation from the data, eleven times. Other nonparametric methods may help [Karlis at al.].
Table 3. Estimates of fraction failing by 36 months
Estimation method | P[Age at failure £ 36 months] |
npmle linear extrapolation | 0.0100 |
nplse linear extrapolation | 0.0100 |
mle Weibull | 0.0079 |
lse Weibull | 0.0094 |
95% mle Weibull UCL | 0.014 |
~90% npmle UCL | 0.027 |
~89.1% npmle UCL | 0.034 |
The variance of the actuarial forecast is (http://www.fieldreliability.com/PrRelAct.htm)
Sn(t)2Var[a(t)]+2Sn(s)n(t)Covar[a(s),a(t)].
This is the variance of E[Failures] = Sn(t)a(t), not the variance of the random number of failures itself. Empirical variance-covariance matrix estimates could be computed from the broom chart estimates, jackknife, or the inverse of the Fisher information matrix (table 4). Those estimates also are necessary for simulating or approximating the distribution of the random number of failures, for estimating prediction intervals.
Extrapolation Uncertainty
Linear extrapolation of nonparametric failure rate functions is a flexible method. Scaled failure rate functions from comparable components that have been in the field longer could be used to help calibrate extrapolation [George, 2003]. Companies (should) have complete warranty failure data for older, similar components to indicate whether linear or other extrapolation of the nonparametric failure rate function is appropriate. Which extrapolation? Weibull himself suggests simplicity, for power function extrapolations, including linear. Bathtub curves show linearly increasing wearout rates, perhaps with justification. Linear extrapolations of the nonparametric failure rate estimates are larger than the maximum likelihood Weibull failure rate.
“To assess uncertainty, make empirical estimates to establish prediction intervals” [Armstrong]. That means, rely on observations or be guided by practical experience and not theory, if you can. Quantitative financial analysts use scenariosto evaluate effects of unobserved but plausible future events. Scenarios are sets of alternative extrapolations.
Extrapolations could be:
- From different data subsets, sequentially as if collected monthly or jackknife
- Other functional forms, not necessarily linear
- Smoothed or grouped data
- Supplemental data from comparable products that have been in the field longer
Linear extrapolation doesn’t capture the apparent alternation of the monthly failure rate function estimates. The alternation has two-or-three-month periods, so it is not obvious how to extrapolate. Quarterly failure rate estimates are smoother but not linear (figure 3).
Financial reporting is usually done quarterly and inventory costs discourage adjusting safety stocks too often, so it may not be necessary to extrapolate monthly alternation. It may be wise to extrapolate the slope as increasing with age (figure 4).

Wallodi Weibull says, “Any distribution can be written in the form 1-e–j(t).” “The most simple function [j(t)] satisfying this condition is…” a power function (t/a)b. The only merit of this df is to be found in the fact that it is the simplest mathematical expression of appropriate form…” Therefore, it seems useful to extrapolate failure rate functions of the form tb-1, so linear extrapolation is equivalent to b = 2. Other reasonable values of b may be used to model extrapolation uncertainty.
The prediction sensitivity to extrapolation parameters helps quantify extrapolation uncertainty. The derivatives of an actuarial forecast Sn(t)a(t) with respect to the intercept and slope of linear extrapolation are Sn(t) and Stn(t), where the sums are over the extrapolation months. The delta method approximates distributions from sample variance-covariance of the linear regression coefficients, http://en.wikipedia.org/wiki/Delta_method. Other uncertainty scenarios can be constructed using subsets of 12-months data a la jackknife.
The inverse of the Fisher information matrix gives a lower bound on the asymptotic variance-covariance matrix of maximum likelihood estimators themselves (under some conditions [Patilea and Rolin]). This inverse helps estimate approximate confidence intervals on actuarial forecasts and the distribution of future failures (prediction intervals). Table 4 is derived from the asymptotic variance-covariance matrix for quarterly nonparametric estimates of the discrete probability density function.
Table 4. Standard deviation and correlations of nonparametric probability density function estimate
Quarter | 1 | 2 | 3 | 4 |
1 | 0.00008 | |||
2 | -0.65599 | 0.00012 | ||
3 | 0.00000 | -0.46790 | 0.00015 | |
4 | 0.00000 | 0.00000 | -0.47099 | 0.00019 |
Sample uncertainty in actuarial failure forecasts and future failure predictions is induced by random variation in the first year’s data. Table 4 represents that sample uncertainty. Maximum likelihood estimators are asymptotically normally distributed (under some conditions [Patilea and Rolin]), so it is reasonable to assume the actuarial forecast and demands are too.
However, variances and covariances of actuarial rates have to be extrapolated, to represent extrapolation uncertainty in confidence and prediction intervals on failure forecasts and future failures. It seems reasonable to extrapolate standard deviations and correlations of table 4 in the same way the actuarial rates were extrapolated.
The Delta method, prediction intervals from linear regression extrapolation, and confidence limits computed from extrapolated variance-covariance matrix are alternatives to represent extrapolation uncertainty. If they agree tolerably, there is some hope that they actually represent uncertainty induced by extrapolation. Then one must hope that the future resembles the past. Information from the first 12 months should be supplemented with full warranty failure rates from other, older, similar components. “Update extrapolation models as new data are received” [Armstrong].
Conclusions and recommendations
Preserve all the information in data, represent sample uncertainty without unwarranted assumptions, and use alternative scenarios to quantify extrapolation uncertainty.
Goodness of fit is a poor reason for assuming Weibull or other reliability function, when nonparametric alternatives are available. Nonparametric estimates fit better. For component D, the Akaike Information Criterion is the same for the 2-parameter Weibull and the 12-parameter discrete nonparametric reliability function. The Weibull assumption added 6.7% uncertainty to the nonparametric entropy estimate.
The maximum likelihood and least squares nonparametric estimators from left-and-right-censored failures are easily computed. Their extrapolated failure rates exceed those of the Weibull failure rate estimates. The nonparametric actuarial forecast is easily computed, and its sample standard deviation is too, with linear failure rate extrapolation for the oldest survivors. The nonparametric actuarial forecast, with a linearly extrapolated failure rate, exceeds the Weibull actuarial forecast substantially. The fraction failing within 36-month warranty and its upper confidence limit do too.
The distribution of future failures during warranty is computable and simulatable from the nonparametric estimates and extrapolations. Future failures are sums of independent and binomially distributed failures from each month’s survivors. Don’t assume Poisson, negative binomial, or normal failure distributions. Simulate future failures = Sn(t)a(t) from binomial failure distributions from n(t) survivors (trials) with conditional probabilities of failure a(t) in the forecast calendar interval.
Contact me, pstlarry@yahoo.com, if you would like nonparametric estimation, forecasts, simulations, and prediction intervals for left-censored failures.
Acknowledgments
The ASQ Quality Progress Statistics Roundtable article contained the data in table 1: 12 sets of installed base and failures. Meeker, Doganaksoy, and Hahn deserve credit for publishing their data. “Including sufficient data and information, so that the readers could duplicate the results if they had the time, inclination, and money, is a sine qua non of scientific publication.” [Heard at a Biostatistics conference a long time ago: still relevant, [Baggerly and Berry]]
References
Armstrong, J. Scott, “Extrapolation for Time-Series and Cross-Sectional Data,” Principles of Forecasting, Kluwer, Norwell, MA, 2001, http://www.forecastingprinciples.com/paperpdf/extrapolation.pdf
Baggerly, Keith A. and Donald A. Berry, “Reproducible Research,” Amstat News, Issue #403, Jan. 2011, pp 16-17
Brown, Wilbur K. and Kenneth H. Wohletz, “Derivation of the Weibull Distribution Based on Physical Principles and its Connection to the Rosin-Rammler and Lognormal Distributions,” J. Applied Physics, Vol. 78, No. 4, pp. 2758-2763, Aug. 1995, http://www.ees1.lanl.gov/Wohletz/SFT_Wiebull.pdf
Çinlar, Erhan, “On a Generalization of Gamma Processes,” J. Appl. Prob., Vol. 17, pp. 467-480, 1980
George, L. L., Credible Reliability Prediction, ASQ Reliability Division, 2003, http://www.asq.org/reliability/quality-information/publications-reliabilty.html
George, L. L. “Broom Charts,” ERI News, Vol. 7, May 2002 and ASQ R&M Tech Briefs, Vol. 1, No. 1, Oct. 2006, http://www.equipment-reliability.com/newsletter/news7/nl7.htm
George, L. L., “User Notes for the Modified Turnbull Reliability Estimate,” unpublished, 2001, revised 2008. Contact me if you want a copy of the notes and the workbook.
Karlis, Dimitris and Valentin Patilea, Confidence intervals of the hazard rate function for discrete distributions using mixtures, Computational Statistics & Data Analysis, Volume 51, Issue 11, Advances in Mixture Models, 15 July 2007, Pages 5388-5401
Meeker, William Q., Splida/RSplida reliability analysis software, http://www.public.iastate.edu/~splida
Meeker, William Q., Necip Doganaksoy, and Gerald J.Hahn, “Predicting Problems: Forecasting the number of future field failures,” ASQ Quality Progress, Statistics Roundtable, Nov. 2010, pp. 52-55, http://asq.org/qualityprogress/departments-columns-current/?column=20&mode=nav&lst=hp
Meier, Paul et al., “The Price of Kaplan-Meier,” J. American Statistical Association, Vol. 99, No. 87, 2004, http://goliath.ecnext.com/coms2/gi_0199-1252501/The-price-of-Kaplan-Meier.html
Miller, Rupert G., Jr., “What Price Kaplan-Meier?” Biometrics, Vol. 39, pp. 1077-1081, Dec. 1983, http://www.scharp.org/users/yqchen/class/Miller1983Bmcs.pdf
Newman, W.; Turcotte, D. L.; Shcherbakov, R.; Rundle, J. B. “Why Weibull?
“American Geophysical Union, Fall Meeting 2005, abstract #S43D-07, http://adsabs.harvard.edu/abs/2005AGUFM.S43D..07N
Patilea, V. and J.-M. Rolin, “Product-Limit Estimators of the Survivor Function with Left or Right Censored Data,” IAP Statistics Network, Tech. Report 0511, http://www.stat.ucl.ac.be/IAP
Weibull, Wallodi, “A Statistical Distribution Function of Wide Applicability,” ASME J. Applied Mechanics, Vol. 18, No. 3, pp. 293-297, 1951
Discussion of “Nonparametric Forecasts…” Article
Ernst Hjalmar Waloddi Weibull
Dec. 13, 2010
It is gratifying to see the developments in statistics and reliability since I died, particularly the use of the Fréchet distribution that I described in my 1939 and 1951 papers. It is now widely referred to as the Weibull reliability function. It seems to be overused in some subject areas, without careful reasoning and justification. It is, however, a better representation of reliability than a constant failure rate.
It is not gratifying to see how the author has abused Weibull statistics in an earlier article to recommend mixing bad, infant mortality units with good units to achieve a specified MTBF according to the Weibull mixture mean, E[Life] = aG(1+1/b1)/h1+(1-a) G(1+1/b2)/h2 where b1<1<b2 [George 1998]. Sadly, that author persecutes engineers who use Weibull software without first plotting and understanding their data, http://www.fieldreliability.com/VirtStat.htm. That author uses my own words to justify the power law extrapolation of nonparametric failure rate functions.
Engineers must decide whether to extrapolate nonparametric failure rates or use the Weibull failure rate function beyond the age of the oldest observed failure. Engineers must make sure that anomalies in data collection do not corrupt parameter estimates and forecasts before accepting the Weibull or other extrapolations.
Except for extrapolating actuarial failure rates for quantifying uncertainty, the author’s paper contains nothing new. In fact actuarial failure rates and forecasts predate my 1939 paper. As I wrote in my 1951 paper, “the Weibull distribution has no theoretical basis…there are-with very few exceptions-the same objections against all other df… It is believed that in such cases the only practicable way of progressing is to choose a simple function, test it empirically, and stick to it as long as no better is found.”
Has the author seen or heard of “Probabilistic Risk Analysis” (PRA)? PRA caused me to spin in my grave. PRA is the deliberate randomization of distribution parameters, such as those of the Weibull reliability function, to represent uncertainty. Here in physics heaven, we know the joint distributions of such random parameters, but you mortals don’t seem to have a clue, yet you randomize one or two independent parameters conveniently to represent uncertainty. That’s a good way to increase uncertainty. Of course, up here we also have no need for forecasts or predictions.
George, L. L., “What MTBF Do You Want?” ASQ Reliability Review,
- Weibull, (1951), “A statistical distribution function of wide applicability”, J. Appl. Mech.-Trans. ASME 18 (3): 293–297
GE Consumer & Industrial Appliances
Richard Zutterman
National Service Manager
Consumer Home Services
Appliance Park, AP6-239
Louisville, KY 40225
USA
T 502-452-3168
F 502-452-7940
November 7, 2006
Dear GE Customer Care Servicers,
GE Consumer & Industrial places a very high priority on product quality and customer satisfaction and thus continuously assesses our product reliability. Recent analysis has discovered a potential problem with the main circuit board on a limited number of refrigerator models, which could lead to lower than anticipated life expectancy on that component. Therefore, if the main circuit board fails, GE Consumer & Industrial will replace this part free of charge to the consumer until further notice.
Models Covered: see attachment
The models affected are side-by-side plastic liner, side-by-side metal liner, bottom freezer two door and bottom freezer French door. The models were produced between May 2005 and August 2006 (HH – RL serial range) and are included in this program. See enclosed list.
Potential Additional Covered Parts:
Specific parts involving the failure of the main board are included in this program. These parts are limited to the following:
-Evaporator Fan failure, only if accompanied by a Main Board failure
-Dispenser Board failure, only if accompanied by a Main Board failure
The main board part number in cases in WR55X10552. Please use your normal warranty MA00 job code for this repair. If you encounter an evaporator fan or dispenser board failure due to the above main board, please include on your invoice and use the normal MA00 job code. It is critical that you verify the proper functioning of the unit including the evaporator fan motor and dispenser board before leaving the home to avoid a repeat call.
Units may be in or out of the standard 1-year product warranty and both instances are covered under this concession program.
If you previously completed this repair and charged the consumer, please send a copy of the service invoice to General Electric Consumer Relations, PO Box 10736, Norfolk, VA 23513 or fax a copy to 1-877-299-0725 so that we may issue a refund to the consumer.
Sincerely,
Richard Zutterman
Postscript, Dec. 21, 2010
I thought component D could be the GE refrigerator motherboard, WR55X10552, http://applianceguru.com/forum1/13243.html. Our GE microwave control board WB27T10091 is starting to fail, again. It is of the same vintage, part of a microwave-oven model JKP86BOF3BB installed April 2005. At least two other microwave-control board failures are on the Internet. Our first control board was replaced in Sept. 2010. The repairman was not surprised, but the control board was not stocked. The only such board stocked is the refrigerator motherboard WR55X10552. I wonder if there is a common cause? [llg]
Postscript, Jan. 3. 2011
Bill Meeker says, “First, I need to point out that Component D was not from a GE product. When I have been involved in prediction problems in the past (for a variety of companies and products), I have sometimes been able to get permission to use the example and data by not using the company or product name. In some cases (e.g., in my May 2010 Technometrics article with Hong) I also had to simulate data so we could at least present the methodology.” I apologize and wonder what component D is? [llg]
Leave a Reply