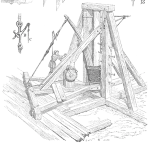
Most manufacturers would rate product quality as a key driver of their overall ability to satisfy customers and compete in a global market. Poor quality is simply not tolerated. It follows that manufacturers require objective measures of their product quality. While many companies still think of quality as “being in specification,” progressive companies focus on reducing variation to minimize waste and produce products that perform consistently well over time. Quality may be thought of as inversely proportional to variation–that is, as variation increases, product quality decreases.
Since variation and quality go hand-in-hand, we require objective measures of variation. The standard deviation is a statistic that describes the amount of variation in a measured process characteristic. Specifically, it computes how much an individual measurement should be expected to deviate from the mean on average. As shown below, the larger the standard deviation, the more dispersion there is in the process data.
All three processes above have a mean (average) of 10, but the standard deviations vary. A smaller standard deviation means greater consistency, predictability and quality.
We can define a population (or process) standard deviation (usually indicated by σ) as well as a sample standard deviation (usually indicated by s). Typically, the true process standard deviation is unknown so we compute a sample standard deviation in order to estimate it.
Computing the Standard Deviation
The formula for computing the standard deviation depends on whether we are computing the population (process) standard deviation or the sample standard deviation. In order to compute the true value, all of the data comprising the population or process of interest must be measured (usually not feasible).
The formula for computing the true process standard deviation is:
$$ \displaystyle \sigma=\sqrt{\frac{\sum_{i=1}^{N}\left(X_{i}-\mu\right)^{2}}{N}} $$
where Xi = the ith data value, μ = the true process average, N = the population size
Essentially, the formula tells us to do the following:
- Compute the process average μ
- Subtract the process average from each measured data value (the Xi values)
- Square each of the deviations computed in step 2
- Add up all of the squared deviations computed in step 3
- Divide the result of step 4 by the sample size
- Finally, take the square root of the step 5 result
Why do we need to square the deviations then take the square root? If we simply computed the average deviation of each value from the mean (the step 2 values)–we would always get a result of zero! (This is because the positive deviations would cancel the negative deviations.) Squaring and “un-squaring” resolves this issue and ensures that our standard deviation will have the same units that our measured values have.
An example: My daughter’s soccer team has eight girls on the team and their heights in inches are as follows:
46.3, 48.4, 47.1, 45.8, 48.0, 50.1, 46.7, 48.5
The standard deviation of the team’s height (we have access to the entire population here!) is found using the above formula and steps:
- Compute the process average μ
$$ \displaystyle \mu=\frac{46.3+48.4+47.1+45.8+48.0+50.1+46.7+48.5}{8}=47.6 $$
- Subtract the process average from each measured data value (the Xi values)
- Square each of the deviations computed in step 2
- Add up all of the squared deviations computed in step 3
- Divide the result of step 4 by the sample size
- Finally, take the square root of the step 5 result
$$ \displaystyle \begin{aligned} \small \sigma=&\sqrt{\frac{(46.3-47.6)^{2}+(48.4-47.6)^{2}+(47.1-47.6)^{2}+45.8-47.6)^{2}+(48.0-47.6)^{2}+(50.1-47.6)^{2}+(46.7-47.6)^{2}+(48.5-47.6)^{2}}{8}}\\\sigma=&1.4 \end{aligned} $$
The Sample Standard Deviation
Usually, we can only estimate the true standard deviation by using a sample. The formula for a sample standard deviation (S) is slightly different than the formula for s. First of all, since we cannot compute μ (a true population or process average), we must estimate it using the sample data. This is called the sample average and is usually called x-bar. The other difference is not so obvious. Rather than dividing by the sample size (N), we divide by (n-1). The formula is:
$$ \displaystyle \textrm{S}=\sqrt{\frac{\sum_{i=1}^{n}\left(X_{i}-\bar{X}\right)^{2}}{\left(n-1\right)}} $$
where Xi = the ith data value, x-bar = the sample average, n = the sample size.
The reason for dividing by n-1 has to do with a concept called “degrees of freedom.” (An intuitive explanation will be given in a future article.)
Uses of Standard Deviation
Many statistical methods such as Statistical Process Control, Gage R&R, and Design of Experiments utilize the standard deviation to estimate variability. Sometimes the square of the standard deviation (called the Variance) is utilized because of its nice additive properties.
The key point is that the standard deviation is an objective measure of variation. Focusing on minimizing the standard deviation of key process characteristics will result in higher quality and customer satisfaction.
Leave a Reply