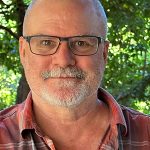
A Discussion on Weibull Analysis with Fred Schenkelberg
This episode of the weekly podcast discusses the basics of Weibull analysis and distribution technique. By using it you can precisely calculate the probability of failures through a statistical analysis of the failure data. As it is a statistical way of calculating the failure rate and getting an idea about the downtime and life of the equipment, it needs data.
This data is then plotted in the form of a graph whose slope values tells us about the results of our analysis and gives us an estimate about issues and problems that can arise. The widely used software for Weibull analysis is Weibull++. There are many alternatives available for this purpose and one of the good ones is Minitab which works same as Weibull++. There are multiple columns available for each formula available in the package. You have to enter these initial values and the formula takes care of the plot after calculating the slope of the graph. This slope value is then used in calculating the arithmetic means, variations, and regressions of the input data.
The basic features of Weibull are,
- It tells us about the life of the equipment by analyzing the input reliability data obtained from different sources.
- This data is then distributed using different models available in the package library of the software under different failure modes such as exponential, normal, Betta, Gemma and so on.
- There are tools that run reliability and warranty tests. Then, calculate the results using the probability function. The shape is determined by the Betta value in the lifetime function represented by f(t).
- These results are then plotted on a graph which has probability failure values on the Y-axis and life-time failures of the equipment on the X-axis. This is called a 2-parameter Weibull distribution. The slope of these parameters tells us whether the assumptions were Weibull or not. The shape of this plot gives us information about our data flow.
- These results then formulate a statistical reliability data analysis report. This final report then tells the reliability management department about the performance of their equipment in its warranty period.
This Weibull++ software helps you then in forecasting a lot of things such as,
- How will the equipment perform during its life-time?
- Whether an item meets the specifics of the reliability requirements or not?
- To what extent the results differ from the manual provided by the OEMs?
This analysis may not be as effective as RCM or FMEA. But, it saves money and time that is consumed in performing such detailed reliability checks. That is why Weibull probability analysis is used extensively for better estimation of failure rates and required maintenance calculations. But, for getting precisely accurate results, failure data needs to be collected from all the possible sources. This keeps the graphical result line as straight as possible, because more values mean, more points on the graph which makes it easy to calculate the approximately correct results.
References:
- Weibull Distribution (article)
- The New Weibull Handbook
- Accendo Reliability
- Fred Schenkelberg
- www.Reliability.FM
- FMS Reliability
- Weibull++ by Reliasoft
- Minitab
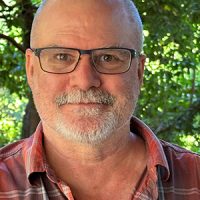
- Social:
- Link:
- Embed:
Rooted In Reliability podcast is a proud member of Reliability.fm network. We encourage you to please rate and review this podcast on iTunes and Stitcher. It ensures the podcast stays relevant and is easy to find by like-minded professionals. It is only with your ratings and reviews that the Rooted In Reliability podcast can continue to grow. Thank you for providing the small but critical support for the Rooted In Reliability podcast!
Hi, James/Fred
I got a question, How can i performe a weibull analysis in population that have already 5 years opertating but any failure data was colected in the first two years, only failure data for the last 3?
Thanks
Hi Alfredo,
Yes you can and given you know when the items went into service, and I’m assuming you know the time to failure for each failure, you can determine the time to suspension as well. For example if item one has run for 2000 hours to date and hasn’t failed, it is suspected at 2000 hours. Another item may have run for 1450 hours then failed. It was replaced and that item hasn’t failed yet… say the replacement went online 600 hours ago, this third item is suspended at 600 hours.
The NIST article explains a bit about the weibull distribution and includes code fro dataplot and R software, both free packages, that can help you find the fitted parameters for your data.
http://www.itl.nist.gov/div898/handbook//apr/section1/apr162.htm
Other packages can also do the regression analysis, such as Weibull++, JMP and others.
Cheers,
Fred
Thanks Fred…!!!!
The main problem is that we do not have the time to failure for those happennig in the first two years, we know the time they were placed in service but no failure record was taken, just at the beginnig of the year 3 the company start taking formal notes about failures. And know we want to know the failure modes beheviour and if the Beta value is changing.
Regards
Hello James and Fred,
I am working on a project that compares Minitab Warranty analysis with Reliasoft Weibull Warranty analysis.
For Minitab, I used the Pre-Process Warranty Data tool and changed my end time to represent right-censored data. I then used the Warranty Prediction tool (Weibull Distribution and Maximum Likelihood Estimation Method).
For Reliasoft, I entered the same exact Nevada chart. Allowed returns at time =0, a distribution of 2P-Weibull, MLE, Standard ranking method, and Kaplan Meier.
From both of these applications, I received a different beta and eta (Minitab Beta = 1.179 and Eta=135.77) (Reliasoft Beta=1.118 and Eta=150.56). I am not sure what I am doing wrong, I am hoping that you would know? Or could explain why one application is better than the other?
Hi Chanel,
Even is the basic settings are the same, I’ve found that each software package for data analysis uses slightly different algorithms. Years ago a few of us ran the same dataset for a Weibull fit and found no two packages agreed exactly. Close, yet not the same.
Without the data nor both of the packages you mention it would be difficult for me to run the analysis as a double check.
One thing you can do is check the parameter confidence bounds – I would not be surprised if both packages have enough of a bound to include the other package’s results.
One other way to check the packages, at least in Weibull++ is to set the beta to the value found in the Minitb result and see if Weibull++ fit the curve with the same eta value.
Overall the two results are similar, as least based on my limited information about the data.
Cheers,
Fred