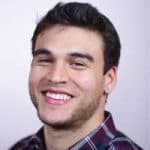
Empowering RES with Automation
Industry 4.0 is here to stay. Organizations are now getting their feet wet in Artificial intelligence and Machine Learning as a way of increasing their output. However, aa good number of these companies have their projects stuck at the pilot stage, either due to inadequate management support, or simply communicating value to other departments. AJ Alexander walks us through the understanding of why this is a good time to start pilots and stimulate their growth.
Important highlights include:
- Understanding the type and stage of data for Machine Learning
- The advantages of successful ML deployments
- Why organizations are slow to adapt
- Hw can you go about increasing the chances of transitioning from pilot to project
What type of data is there for use?
Today in industry, there can be a variety of data streams. These can range from process data to master data and even real time data. Machine Learning (ML) algorithms can work on any type of these data streams, the only condition being that the data is numerical and ith a time series. Examples of this data could be positioning, centering, temperature, pressure, humidity to name a few
Where the information gets stored
Information from processes and equipment can get stored in either proprietary servers or with other known cloud services platforms like Google and Amazon. As long as the data collection has been designed by people, it can be stored and modeled.
Can organizations leverage ML expertise to make an improvement?
The answer is yes. A data scientist’s main job is to take your data sets and structure them in a way that teams and model, or use it to train algorithms. Organizations with a process engineer have a slightly easier time because this is the person with an understanding of the process. S/he can also help prepare data for the data scientist.
Today, the available machine learning tools are also becoming more affordable, user-friendly and robust. Examples are Sorba and Open Protocol IoT which have a drag-and-drop approach to building models.
Types of decisions that this expertise allows us to make
Artificial Intelligence (AI) and Machine learning deployments mainly accelerate detection and prediction needs for organizations. More measurable results of these technologies can be increased uptime, condition monitoring of equipment, failure detection, root cause analysis, etc.
Why don’t integrators take advantage of ML?
Traditionally, tech companies would sell a box or a disc as a solution to a business. Customers were limited by the provisions of the presented hardware and software capabilities. That is shifting today.
As customers have unique processes and assets, it now becomes a dynamic role for integrators to tweak solutions almost on a project basis. This need also means that integrators need to team up with the domain experts so as to deploy successful ML projects.
Why organizations are still slow on adoption
With any technology change, cultural change is one of the most significant determinants of success. For industry, departments that act as silos add a layer of difficulty to adoption. There has to be a communication and operational link between Information Technology (IT) and Operations Technology (OT) departments.
Top management buy-in is also necessary for the adoption of these programs. Once the product champion has received management backing, it then becomes their role to win the support of the other staff.
Technologies available for implementation of ML
The domain of Machine Learning contains a wide range of libraries that can be applied to facility data. Examples are Neural Networks which facilitate training and prediction algorithms.
What makes the biggest difference
To realize the potential of technology projects like this, communication through the organization is key. The product champion must work to demonstrate the importance of these technologies to siloed departments. Otherwise, the pilot project might never grow to make an impact in the organization.
Key takeaways
The main learning points from this episode are that:
- Machine Learning deployments are heavily based on a clear problem definition. Therefore ensure that the team in charge has involved various departments in the organization to get an appropriate description of the problem.
- Communicate your intentions continuously and seek input across the organization. This approach also helps people buy into the project as they are being involved.
- Surrounding yourself with domain experts also ensures that they can help you refine your design based on the field realities.
What people can do today
The key takeaway is to continue learning, asking questions and deploying demos. Starting small gives you validation early on on the success of ML deployments before scaling up.
Eruditio Links:
AJ Alexander Links:
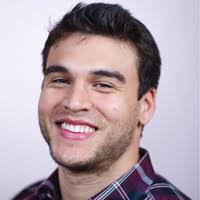
- Social:
- Link:
- Embed:
Rooted In Reliability podcast is a proud member of Reliability.fm network. We encourage you to please rate and review this podcast on iTunes and Stitcher. It ensures the podcast stays relevant and is easy to find by like-minded professionals. It is only with your ratings and reviews that the Rooted In Reliability podcast can continue to grow. Thank you for providing the small but critical support for the Rooted In Reliability podcast!
Leave a Reply