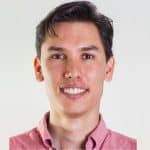
Am I ready for AI with Geraldo Signorini
We’re pleased to have Geraldo, the principal consultant at Eruditio. He spends a lot of time doing reliability activities like instructing and consulting, among others. He started his engineering career in 2008 and spent more than ten years in oil and gas in roles related to reliability and maintenance engineering. He’s also talked at presentations and contributed to articles on Machine Learning and Artificial intelligence, which is the topic we’ll dive into.
In this episode, we will be discussing:
- What is AI or ML
- More prerequisites to prepare for AI or ML
- What are the roles you need in place for AI or ML
- Are any more fundamentals necessary
…and so much more!
What is AI or ML?
There are several different definitions and approaches to answer this. However, Geraldo defines it as a concept that means a machine will be able to make decisions and adapt to the environment it’s in, enabling it to create influence for better decision making with the given parameters it measures. However, most people talking about AI actually mean Machine Learning. This is a set of algorithms and techniques performing data analysis to identify patterns and relationships to create insights that lead to better decisions.
It’s basically trying to teach computers to make better decisions.
How do you know if you’re ready for ML or AI?
Most tasks dedicated to assets are related to data-driven decisions. However, you’ll find that more than 90% of the data plants and industries generate doesn’t get used. So, are you using data to make decisions? Besides KPIs, that includes any data that your machines, facilities or even work orders and the data technicians generate.
So, to know if you’re ready:
- Start by identifying the data you want to utilize
- Assess the data quality
From that point, you’ll need to check data:
- Consistency
- Accuracy
- Completeness
- Auditability
- Orderliness
- Uniqueness
- Timeliness
More prerequisites to prepare for AI or ML
You need to understand the physics of failure of what you’d like to analyze. Then, to complement the quality of the data, you need to understand the asset’s functionalities.
What are the roles you need in place for AI or ML?
You don’t need to have everything to start. Worrying about creating a whole structure from the beginning will wind up wasting time. Have the engineers that help you with solving day to day issues or perform the Root Cause Analysis and such tasks to look into the data. Use the basic statistical tools available to help them analyze how the data behaves before a failure occurs.
Most of the insights and knowledge to start an AI project comes from failure analysis in the fail investigation phase. You need a person that knows the equipment to be able to translate that to the algorithm you want to build. So, the model for your AI or ML project to be able to identify failures or to help make better decisions will come from the knowledge that you build.
The roles you would need to get started would be the ones you already probably have. That includes the engineers, technicians and subject matter experts you have in your facility. They are in the best position to start bringing insights. Once you’re ready to scale up, you’ll need knowledge and understanding of programming languages and such to enable you to build your model.
Are any more fundamentals necessary?
Without fundamentals in place, scaling up later becomes difficult. The fundamentals of reliability and maintenance engineering in terms of hierarchy, criticality analysis, a good FMEA or RCM can give you an easier way to look into this project and automate it later on. They make sure you understand what you’re doing and what you’re looking for.
Examples of where AI or ML has been deployed successfully
The concepts of AI have been around since the 40s and 50s. Alan Turing is considered as the father of computer science, and he proposed the first question on whether a machine can think. Seventy years later, we’re still trying to understand the best process to follow to answer that question.
There are several successful cases, such as fault finding and failure identification in the oil industry. Some projects Geraldo has worked on have produced 75% – 80% accuracy on failure identification, with at least a week or two of advance predictions on the failures. It also applies in areas like parts usage. You can use AI or ML to help you know when to buy parts based on the parameters and sensors in the facility. In mines in Brazil, the availability of carts and trains got increased by 90%. That’s by analyzing the carts and deciding whether they need to get cleaned before they head back to the mine.
One of the fundamentals you need to understand is what is your problem and how to formulate it. Your accuracy on that will help you define which data is necessary, before you work backwards to look at the data, data quality, and physics of failures, among others.
Do companies have to develop their own ML algorithms, or can they purchase readymade options?
It’s a challenge for every company to have SMEs or personnel dedicated to a single project. Often, you’d want that person to use their knowledge to support different projects at the same time. These days, sacrificing one of your SMEs or specialists to create something from scratch isn’t necessary.
Different platforms are ready to use, like Azure, Google Cloud, and Watson. There are also companies providing data scientists or subject matter experts to help you, as a service, with your project development. The industry is moving towards having specialized service companies that support you in your project.
You don’t have to start perfectly. However, you will become better as you continue. There’s a lot of experts and expertise available that you can leverage on.
What makes ML or AI successful?
- Knowing the physics of the failures
- Know the failure modes that happen the most
- Assess how good your Root Cause Analysis has been
- Assess the quality of your failure investigations
- Recheck the information in your failure reports
What to change about a typical ML project?
The methodology is sound. However, as you get to data collection and data quality assessments and such, it can become overwhelming. The three things that cause most failures are:
- Having a problem that wasn’t properly formulated
- Ensuring you have good data quality
- Understanding the physics of the failure and the knowledge that people have
Geraldo also feels it could be made more fun. He would make it in a way that:
- Creates smaller results
- Has the team participating more
- Gets the data scientists to the field to see how the asset works
Although everything gets done from a machine, about 70% of the knowledge you need is in the field with the asset. 30% is algorithms, data processing and what comes after.
In summary
Get resources from industry leaders that define the fundamentals and applications to create a good project. These will give you insight into the steps you need to become a success at your plant. Then get into statistics books and classes to see that not many software is necessary to process the data. From there, you can move into ML algorithms. You’ll notice a lot of free programming languages like Python that you can start practicing with.
Eruditio Links:
Geraldo Signorini Links:
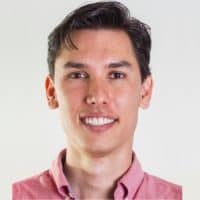
- Social:
- Link:
- Embed:
Rooted In Reliability podcast is a proud member of Reliability.fm network. We encourage you to please rate and review this podcast on iTunes and Stitcher. It ensures the podcast stays relevant and is easy to find by like-minded professionals. It is only with your ratings and reviews that the Rooted In Reliability podcast can continue to grow. Thank you for providing the small but critical support for the Rooted In Reliability podcast!
Leave a Reply