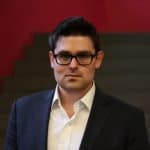
255 – Forecasting the Future with Frank Emery & Stuart Fergusson
Welcome Stewart and Frank to the podcast. Briefly tell us about yourselves
Stewart has a background in Big Data and Applied Mathematics and currently specializes in AI at Fiix. Frank on the other hand has a background in manufacturing of consumer products.
In this episode, we covered:
Our topic of discussion today is around AI and its functionality in forecasting the future and how it helps maintenance professions. Tell us about AI.
AI is basically defined as an algorithm or a series of algorithms that learn and configure themselves based on past activities to predict future behavior patterns.
Why should maintenance professionals care about AI?
The unconventional but appropriate response is that they shouldn’t. AI should be thought of as just another tool which must be evaluated based purely on its functionality.
Its adoption should be assessed while looking at the contribution it makes towards the targeted efficiencies.
Will AI make people lose their jobs?
Negative. If anything, AI is meant to aid professionals in doing their jobs in a better way than they would without it. It assists them in identifying problems much faster and eliminating extended downtime.
What makes a well-rounded AI?
A well-rounded AI is defined by the goals that your organization intends to achieve. It is therefore essential to determine what these goals are before obtaining AI. The tool exists to support the maintenance programs or identify points of asset failure.
Where does all the data used in AI come from?
The data is often obtained from the CMMS already installed. Once you have defined the aspects of operations you want to monitor, the data will be obtained from the tools and systems that you already have.
The data obtained from the tools and systems in place is often inaccurate. How do you deal with this?
The accuracy of data can be suspect but still be useful. If the data is fed into the AI it can show what is being measured and assist in rectifications.
You can improve the quality of the data by perhaps populating more fields to obtain more granulated information from the AI.
However, the key is to determine your goal, the tools being used and the accuracy needed.
How much data do we need to use AI models?
The focus should be ensuring that even if the data is a lot, it ought to be the right set of data. For instance, if you have a data set about a PLC’s performance and another on task scheduling all mixed together, then it will unnecessarily bog down the system without giving data that the AI can optimize.
How do we select what to monitor?
AI looks for anomalies and feeds from sensors to statistically determine which one has too much noise. Clients can also provide contacts for OEMs whom are contacted to help in identifying the sensors to select in an asset.
What are the benefits of AI at the shop floor?
AI has significantly contributed in identifying problems much faster so that maintenance engineers can fix them without extended downtime. The tool also exposes points of highest risk and work order anomalies in perhaps planning the PMs.
Where does one get an AI?
There are a lot of AI software out there. We highly recommend that you do not build one for you own use because it becomes too complex. However, use your problems in searching for a tool that can specifically address them.
What are the successes in AI application?
AI has significantly assisted in flagging work orders. It helped a company in identifying gaps in the work order that ended up saving the entire asset and preventing downtime.
Where can those seeking AI get started?
Chances are that the CMMS you have in place already have an AI option. However, in looking for another one, you have to be specific on what this tool should improve.
The amount of information that it gives should also be easily consumed- AI should not create unnecessary work. It should make the process much easier.
What makes the biggest difference?
The biggest difference is in specifically defining the desired outcomes. This will help in seeking proof of concept and measure results.
Key Takeaway
Do not complicate the acquisition of an AI. It is intended to make things simpler than they already are. If it is too complicated, then it is the wrong tool for your process.
Eruditio Links:
Frank Emery & Stuart Fergusson Links:
- Using WO Data in a Well Rounded PdM Strategy with Frank Emery
- Fiixsoftware.com
- Frank Emery Linkedin
- Stuart Fergusson Linkedin
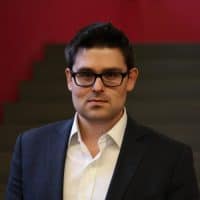
- Social:
- Link:
- Embed:
Rooted In Reliability podcast is a proud member of Reliability.fm network. We encourage you to please rate and review this podcast on iTunes and Stitcher. It ensures the podcast stays relevant and is easy to find by like-minded professionals. It is only with your ratings and reviews that the Rooted In Reliability podcast can continue to grow. Thank you for providing the small but critical support for the Rooted In Reliability podcast!
Leave a Reply