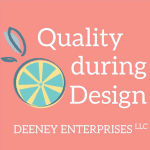
Variable Relationships: Correlation and Causation
Relationships matter! And they can be difficult to understand.
We’re taking relationships between variables. Does one variable affect another? Or do they just correlate? Understanding which matters to the values of our decisions.
We talk about the saying “Correlation does not imply causation”, how to find a confounding variable, and ways to check that we’ve got the triggering cause that’s going to affect our outcome.
When we’re under pressure to figure something out, it’s helpful to stop and remember some fundamentals, like
“Correlation does not imply Causation”
How can we tell that we’re looking at a causal relationship?
- We get consistent results with repeated tests.
- We can replicate the results.
- The results fit general theories, findings, and experiences.
- The cause precedes the effect (we may want to capture timing data when we run tests).
Citations:
A fun website: Spurious Correlations (tylervigen.com)
Other Quality during Design podcast episodes you might like:
Solving symptoms instead of causes?
A big failure and too many causes? Try this analysis.
Episode Transcript
Correlation does not imply causation. We have to keep this in mind every day just from published news articles and studies. It’s also really important for product design, engineering, and well really any engineering at all. Let’s talk more about these relationships between variables after the brief introduction.
Hello and welcome to Quality During Design, the place to use quality thinking to create products, others love for less. Each week we talk about ways to use quality during design, engineering, and product development. My name is Dianna Deeney. I’m a senior level quality, professional, and engineer with over 20 years of experience in manufacturing and design. Listen in and then join us. Visit quality during design.com.
In engineering, we are looking at the relationship between variables all the time. Usually it’s a stress versus strength relationship, the kind of stress that’s a force, a pull, temperature, time vibration, versus our products performance, and it doesn’t have to be a stress, strength relationship either. It could be one thing affecting the other. This relationship between these different variables that we’re assessing every day, they can be just a correlation or it could be a causation. When we’re figuring out the relationship between these variables, if we mistakenly say that it’s a causation when in fact it’s not (it’s just a correlation) that could lead to bad engineering decisions.
Let’s differentiate what is a correlation versus a causation. Correlation means that variables are associated. The values vary together. If one variable increases, the other one increases, or if one variable increases, the other one decreases at the same time. They’re related. We can use correlation if we’re only trying to predict events, if we’re not trying to understand why or get to the root cause of anything. A mistake we can make is assuming that because two variables are correlated, that if we adjust the one, it’s going to affect the other, and that’s not necessarily the case. Now we’re getting into causation.
A causation relationship between variables is when we change one, it ultimately affects the outcome of the other. It’s a cause. One variable is the cause of another variable. When we’re in a situation where we want to affect the outcome, we want to look for a relationship that is a causal relationship.
Let’s look at an example. Our neighborhood families eat more Girl Scout cookies the colder it is outside-the colder the temperature is outside. There is a correlation between those two variables, the colder weather temperatures and eating cookies. When it’s colder outside, we eat more cookies. Just intuitively, we know that one doesn’t cause the other unless you think maybe it’s because we’re all housed up in our homes with nothing else to do but eat cookies. We know that there’s something else going on. When we do some more investigation or thinking about it, we find out that there’s a confounding variable involved. It’s a variable that we didn’t originally look at, but it’s affecting the outcome of the other original two. And that variable is that the Girl Scouts in the area where I live sell cookies to the neighborhood in February and March, which also happens to be the coldest day [months] of the year. This confounding variable could be the cause. The reason why we eat more cookies in February and March has nothing to do with the temperature. Tyler Vigen produced a website and some books called Spurious Correlations. I’ll include a link to it in the blog.
This Girl Scout cookie example, and the examples on the Spurious Correlations website are pretty obvious and honestly a little funny. It’s not so obvious, and definitely not as funny, when we’re looking at variables within our workplace. Within design engineering, whether the relationship between the variables we’re studying is just a correlation or if it’s a causation isn’t always so obvious. Usually in engineering, we want to affect the outcome. We want to make an improvement, change a design, to have a different output. We want a causation relationship between the variables we’re looking at. If we don’t have that or we’re not confident that we have that, then we might start making changes or tweaks and not really getting the outcome that we expect or that we want. We’ll end up spinning our wheels, spending a lot of time on changing these different variables when it’s not going to give us what we really need.
In the end, there are lots of tests that we can do to make sure that we’re working with a causal relationship. When we do tests, we’re going to get consistent results, which means a one-off test to prove something may not be enough. We may need to repeat it a few times. Speaking of repeating, we’re going to be able to replicate the results. We can also rely on our engineering experience and the experience of our team. Do these results fit general theories and findings and things that we already know about what we’re looking at? If it doesn’t make common sense or intuitive sense, or if it flies in the face of anything we’ve seen before, we may not have a causal relationship. Another key thing to look for (which seems obvious after you hear it, but when we’re in the throes of trying to figure stuff out, may not be) is that the cause is going to preceed the effect. The timing of our variables and the effects that we’re monitoring – it matters, and it may be something that we need to capture when we’re capturing the data in our tests.
What’s today’s insight to action? When we are under pressure to solve problems and come up with an answer, we can sometimes forget these fundamentals. When looking at data, determine how it’s related – how the variables are related to each other – before we start making decisions about it. If we’re trying to affect the outcome and get better results, then we really need to make sure that we have a causal relationship and that, ideally, we’re working with a root cause.
If you like this topic or the content in this episode, there’s much more on our website, including information about how to join our signature coaching program. The Quality During Design Journey. Consistency is important, so subscribe to the weekly newsletter. This has been a production of Deeney Enterprises. Thanks for listening!
Leave a Reply