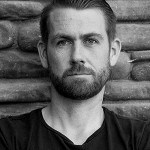
Confidence and Tolerance Intervals
Abstract
Chris and Fred discuss the difference between ‘confidence,’ ‘tolerance’ and ‘prediction’ intervals. Is there any point in understanding the difference between these concepts? … or is it something only statisticians find useful?
Key Points
Join Chris and Fred as they discuss the difference between ‘confidence,’ ‘tolerance’ and ‘prediction’ intervals. Reliability, quality, and manufacturing engineering involve lots of different statistical concepts. So do these concepts help us?
Topics include:
- Tolerance Intervals. Take for example the process of baking bread. Baking bread is a random process. If you are good at baking bread, each loaf should be relatively similar to every other loaf. But there will be some variation from loaf to loaf. This is what makes it random. One characteristic of bread is ‘chewability.’ It is measured in terms of force (Newtons). So if we were to look a 1000 loaves of bread we baked on a particular day, the 95 % tolerance interval of bread chewability will be that interval within which 95 % of our loaves of bread fall. So for example, if 95 % of our loaves of bread have a chewability that is between 6.871 and 7.249 Newtons, then the 95 % tolerance interval (for chewability) is 6.871 to 7.249 Newtons.
- Confidence Intervals. Tolerance intervals are characteristics of bread. Confidence is a measure of you. If we keep looking at our bread, we might be interested in a particular random process characteristic. Like the mean or average chewability. Let’s say that we measure the chewability of one loaf of bread, and it turns out to be 6.945 Newtons. We can use this figure to estimate the mean chewability of each loaf of bread. But how accurate is this estimate? How certain can we be that the mean of every loaf of bread is close to the chewability of a single loaf of bread we measure (6.945 Newtons)? The answer is … not very confident. So if we measure more loaves of bread, and increase the amount of data we have, then we can estimate the mean with increasing accuracy. We can use statistics to actually capture or describe this confidence interval. So the 95% confidence interval on chewability mean will be much wider if we have only measured one loaf of bread.
- Prediction Intervals. This is much like the tolerance interval, but instead it applies to something that is ‘yet to happen.’ We might (for example) be able to calculate the tolerance interval for the 1000 loaves of bread we baked today, and then say (with some justification) that we would expect the same thing to happen tomorrow. There are some circumstances where we might want to do things like change baking temperature or the amount of flour we use, which means that we might be able to calculate the effect this has on the chewability of our loaves of bread tomorrow. So they are not always the same thing.
- One more thing about confidence intervals. Tolerance and prediction intervals technically do not change based on how many samples we have investigated. Confidence does. The more data we have, the more confidence we have. Tolerance intervals (for example) are ‘what they are.’ We just need to get enough data to find them!
- Do these definitions help you? Great! Use them. If not … don’t use them!
Enjoy an episode of Speaking of Reliability. Where you can join friends as they discuss reliability topics. Join us as we discuss topics ranging from design for reliability techniques to field data analysis approaches.
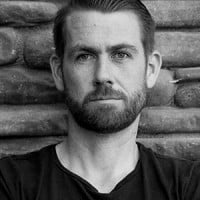
- Social:
- Link:
- Embed:
Related Topics
Reliability Techniques For Analyzing And Improving Fault Toleranc(Opens article in a new browser tab)
Leave a Reply