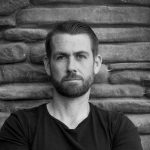
When is MTBF OK?
Abstract
Chris and Fred discuss the MTBF … and if and when it can be used … sometimes in reliability engineering. We know that the MTBF is one of the most chronically overused (and misused) so-called ‘reliability’ metrics. But is there scope for it to be used … sometimes?
Key Points
Join Chris and Fred as they discuss if and when the MTBF can be used. We see it in textbooks and standards. Professors use it all the time. So it is little wonder that students and engineers also seem to rampantly misuse the MTBF and make (sometimes) disastrous decisions. There is nothing wrong with the ‘mathematics’ of the MTBF – it is all about how it needs to be used. But are there times when the MTBF can be used?
Topics include:
- Scenarios where failure occurs with a constant hazard rate. But this is very, very rare. There are plenty of failure modes that have a constant hazard rate where external environmental stresses cause catastrophic failure. Think about tornados, tsunamis, and nails on the road for your car tire. It doesn’t matter how old or young your system is … these failure modes will be equally likely no matter how old or young your system is. But … to find an entire system that has a constant hazard rate is very, very rare. For example, while our car tire will potentially have one ‘puncture’ failure mode that has a constant hazard rate, it will also wear out.
- Scenarios where the MTBF is used to define a probability distribution … in conjunction with another parameter. Like the normal distribution (or bell curve) that models wear out failure phenomenon. But here, we are still not just relying on the MTBF to characterize the nature of failure.
- Logistics and sparing. The Poisson distribution is often used to model how many spare parts we need for a certain interval or duration. It is based on an assumption of a constant hazard rate. We know (through this statistical thing called the ‘central limit theorem’) that if we expect to have a large number of failures, then we know that the Poisson distribution becomes increasingly accurate. Think about 30 or more failures. But if you are expecting only a few spares in an interval … then the Poisson distribution will almost certainly lead you to over-estimate how many spare parts you need.
- Drenick’s Failure Law … asserts that in series systems composed of many components with small failure rates, which are immediately replaced with “good as new” components or perfectly repaired when they fail, system failures will be (asymptotically) exponentially distributed almost regardless of the component failure time distributions. But this doesn’t mean the underlying components are exponentially distributed (which means it only needs the MTBF to define failure behaviour). What this means is that when there is a huge number of components being replaced in this way, we have a ‘perfect’ mix of old and young components. Which means that even though individual failure mechanisms are wearing out, the system appears to have a constant hazard rate. But this takes TIME! And is rarely checked. And doesn’t take into consideration how things like preventive maintenance (PM) ‘reset’s component lives – all at once.
- Accelerated life testing … only where you are comparing two materials. If you have an underlying understanding of the Physics of Failure (PoF), you might be able to compare two materials in terms of their MTBT (or MTTF) only. This might help you make a quick decision on which material to use … but you also need to check that the underlying Time To Failure (TTF) distribution aligns with what you expect if you are going to use this data to predict reliability.
Enjoy an episode of Speaking of Reliability. Where you can join friends as they discuss reliability topics. Join us as we discuss topics ranging from design for reliability techniques to field data analysis approaches.
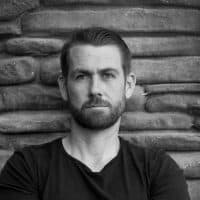
- Social:
- Link:
- Embed:
Show Notes
Leave a Reply